Logility named a Leader in the 2024 Gartner® Magic Quadrant™ for Supply Chain Planning Solutions
Gartner delivers actionable, objective insight to executives and their teams. It...
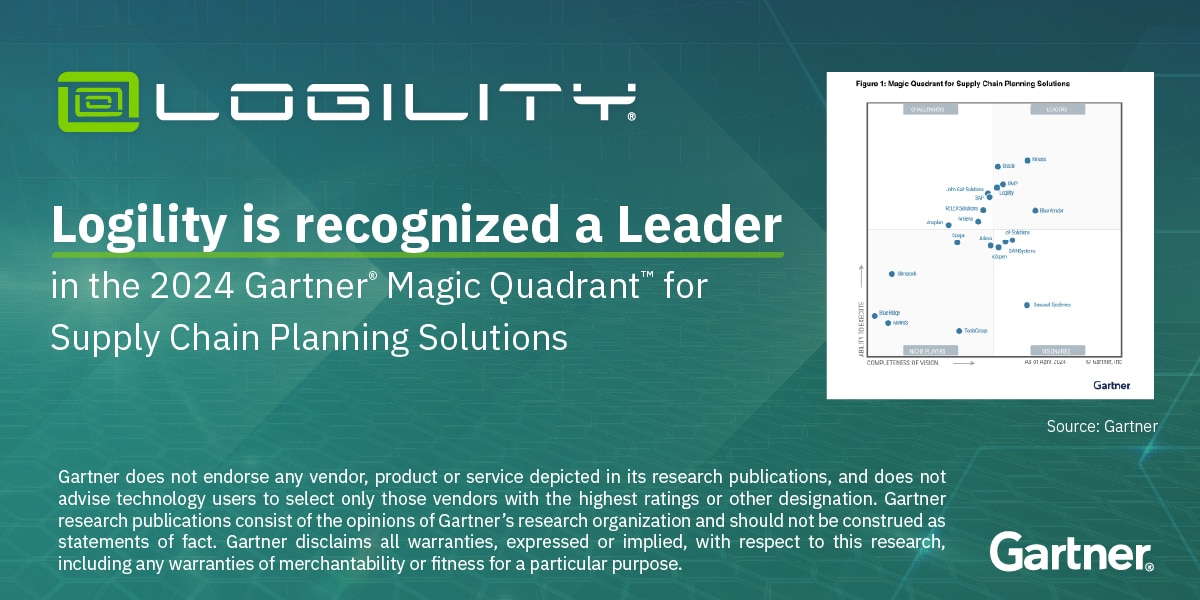
Gartner delivers actionable, objective insight to executives and their teams. It...
In this client testimonial, WAI Global shares how they have forecast accuracy im...
Logility, Inc., the leader in AI-first supply chain planning solutions, today an...
Logility, Inc., a leader in AI-first supply chain planning solutions, today anno...
Planned Reclassification Simplifies Equity Capital Structure and Improves Corpor...
Accelerate Decision Making with a Dose of Digital Transformation Over the last 1...
SGS Maine Pointe leverages Logility’s network optimization solution to drive f...
Bondi Sands embraces technology transformation with Logility to support exponent...
PPC’s S&OP Journey–Leveraging Customer Collaboration for Business Growth
Radial leverages Logility Network Optimization to determine the optimal footprin...
Leveraging AI for Faster, Strategic Decision Making There is a lot of informatio...
Everywhere we look, artificial intelligence (AI) is pushing the boundaries of ev...
Dow and Ngabire have committed their time and talents to positively influence th...
In an ever-evolving technological landscape, new potential explodes in the suppl...
January 2024 Generative AI is still a hot topic in the supply chain. You may hav...
We are back again with the Logility Supply Chain Pulse Newsletter. We hope you f...
AI-first Supply Chain Planning Solutions to Support Aggressive Business Growth A...
Subscription Fee Growth of 9% and Adjusted EBITDA Margin was 16% of revenue from...
ATLANTA – February 21, 2024 –The Board of Directors of American Software, In...
How an AI-First Mindset Is Reinventing Supply Chain Operations AI has captured w...
Food Logistics Rock Stars Award recognizes influential individuals in the indust...
ATLANTA – February 8, 2023 – Atlanta-based American Software, Inc. (NASDAQ: ...
In an era marked by rapid market shifts and evolving business landscapes, precis...
Transformative new capabilities extend AI-first planning solution ATLANTA – Fe...
We are back again with the Logility Supply Chain Pulse Newsletter. We hope you f...
Vendor management, product traceability, and compliance features offer better ri...
What defines a high-performing supply chain? Depending on who you ask, the answe...
ATLANTA (January 22, 2024) – American Software, Inc. (NASDAQ: AMSWA) has d...
How AI in Supply Chain Brings Value to Your Business Supply chain leaders are lo...
Navigating the $5 trillion North American apparel market requires precision, esp...
ATLANTA (January 5, 2024) American Software, Inc. (NASDAQ: AMSWA), a leading pro...
The Logility Platform leverages enhanced AI/ML capabilities to sense, anal...
From harvest to hands, the food & beverage (F&B) industry leaves no room...
In the rapidly evolving world of supply chain management, relying on outdated no...
Supply Chain AI has sparked considerable excitement, shock, and fear over the pa...
Sales and operations planning (S&OP) has been a longstanding practice for bu...
Logility, Inc., a leader in prescriptive supply chain planning solutions, toda...
In a world characterized by market volatility and unpredictability, businesses c...
Subscription Fee Growth of 8% and Adjusted EBITDA Margin was 16% of revenue from...
Industrial durables companies face the perpetual challenge of managing items wit...
Divestment aligns with American Software’s commitment to AI-first supply chain...
Subscription Fee Growth of 8% and Adjusted EBITDA Margin was 16% of revenue from...
Gartner Supply Chain Planning Summit, Phoenix, AZ. – November 16, 2023 –&nbs...
We are back again with the Logility Supply Chain Pulse Newsletter. We hope you f...
ATLANTA – November 6, 2023 – Atlanta-based American Software, Inc. (NASDAQ: ...
We are back again with the Logility Supply Chain Pulse Newsletter. We hope you f...
Why do you need Generative AI in your supply chain? Your voice assistant probabl...
Results from the New Era of Planning to be Showcased at the 2023 Gartner® Su...
Why Network Optimization Is the Key to Consumer Packaged Goods Supply Chain Resi...
For the last decade supply chain leaders and CIO’s have been evaluating when a...
For any organization, “Demand” fundamentally originates through the ebbs and...
Powerful New Offering Optimizes Inventory with Advanced AI and Machine Learning ...
ATLANTA (September 25, 2023) American Software, Inc. (NASDAQ: AMSWA), a le...
ATLANTA (September 21, 2023) American Software, Inc. (NASDAQ: AMSWA), a le...
Supply Chain Software Implementation: A Prescriptive Approach to Optimize ROI&nb...
Even the most seasoned demand planners have struggled against unprecedented chal...
Divestment aligns with American Software’s commitment to AI-first supply chain...
Taming Supply Chain Bullwhips with DemandAI+ Bullwhip effect is a common term us...
Logility Congratulates Rebecca Springett of Croda & Rita Fisher of Reynolds ...
In today’s fast-paced world, demand planning has become increasingly compl...
ATLANTA (September 13, 2023) American Software, Inc. (NASDAQ: AMSWA), a leadin...
Improve your supply chain planning with speed and data for advanced scenarios.
New regulations, consumer demands, and internal pressures are raising the stakes...
The Fast Track to Executive Buy-in and Rapid ROI
When Logility embarked upon the journey with AI-first planning and DemandAI+ we ...
Supply chain disruption, risk, and complexity are frustrating topics in many boa...
In today’s economic climate, supply chain executives face growing pressure to ...
Supply Chain Planning: The Gratifying Career Choice This is that time of the yea...
When the United States had a massive infant formula shortage, families were unde...
Staying in control with Transparent AI Uncertain supply chain conditions due to ...
In a recent webinar, How ChatGPT Changes All Rules of Supply Chain Planning, we ...
How Human-Machine Collaboration Cuts Implementation, Time, Costs and Error
The world of supply chain planning has undergone significant changes in the last...
Logility, Inc., announced it has signed a definitive agreement to acquire Garvis...
In the realm of demand planning, it is essential to understand the differences b...
How AI powered demand planning solutions revolutionize the role of demand planne...
Artificial Intelligence (AI) is all the buzz, and Generative AI, like the widely...
Demand forecasting plays a crucial role in business success, as it helps predict...
Supply chain planning plays a critical role in ensuring operational efficiency, ...
ATLANTA – August 30, 2023 – Logility, Inc., a leader in prescriptive su...
Two-Thirds of businesses do not have a risk mitigation strategy for their supply...
Subscription Fee Growth of 14% and Adjusted EBITDA Margin of 13% in Q1 ATLANTA (...
Your Comprehensive Guide to Production Planning Software The benefits of p...
ATLANTA – August 23, 2023 – American Software, Inc. (NASDAQ: AMSWA) today an...
We are back again with the Logility Supply Chain Pulse Newsletter. We hope you f...
In today’s rapidly evolving marketplaces, businesses need every advantage ...
Smarter, Not Harder: How to Climb Every Peak in Supply Chain Planning Supply cha...
When it comes to implementing supply chain planning and operations solutions, su...
Efficient inventory optimization starts with choosing the best software. It is i...
Disruptions are the norm in supply chain planning leaders. Customer demand and e...
Lets face it, the world is complex and growing more everyday. The list of supply...
During this webinar with Logility partner Anamind, you will gain invaluable insi...
Just think about the last time you brought your car in for service only to hear,...
(but we will!) Selecting a new enterprise technology or supply chain planning so...
Disruptions in supply chain operations are here to stay. They’re increasing in...
In an increasingly connected and dynamic global business landscape, unpredictabi...
How often do you look at your supply chain and ask the question is this Optimize...
In business, time is indeed money, and nowhere is this truer than within the int...
Minimizing supply chain costs and maximizing sales revenues during these remarka...
Supply chains large and small are under siege by constant supply chain disruptio...
Sales and operations planning (S&OP) was created way back in the 1980s. But ...
Good forecasting leads to good demand planning —and good demand planning means...
We are back again with the Logility Supply Chain Pulse Newsletter. We hope you f...
AI, Artificial Intelligence, is a word being thrown around these days to garner ...
Störungen in der Lieferkette haben zu einer großen Unsicherheit in Bezug auf A...
3PL Selection—don’t let your people be penny wise and pound foolish Are you ...
Think you don’t understand supply chain? Believe it or not, you do it all t...
ATLANTA, GA – July 10, 2023 – Logility, Inc., a leader in prescriptive suppl...
Supply chain planning has become a critical factor for organizations aiming to g...
Minimizing supply chain costs and maximizing sales revenues during these remarka...
A good logistics strategy will move material by the most economic modes within t...
S&OP and Multi-Horizon Integrated Business Planning Manual supply chain p...
Picture this: You’re a seasoned sailor navigating uncharted waters. You...
The first fully web deployed, SaaS Supply Chain Design Service – Logi...
The Logility® Digital Supply Chain Platform increases agility and sustainabilit...
We are back again with the Logility Supply Chain Pulse Newsletter. We hope you f...
Minimizing supply chain costs and maximizing sales revenues during these remarka...
In the constantly changing world of logistics and manufacturing, “what if&...
If you’re just starting your journey of supply chain sustainability, you may b...
The 7 impacts of Supply Chain network optimization In today’s globalized e...
The Gartner Supply Chain Symposium/Xpo conference brings thousands of supply cha...
Top 3 Demand Forecasting Mistakes —How To Avoid Them with Demand planning...
How to properly optimize your logistics network with network design. The Challen...
In the intricate world of supply chain management, the accuracy of demand foreca...
The Optimization Advantages of a Redesigned Supply Chain Network Cost optimizati...
ATLANTA — May 31, 2023 — Logility, Inc., a leader in prescriptive supply cha...
Multi-horizon integrated business planning with robust scenario analysis is a cr...
In today’s fast-paced and unpredictable global market, the secret to maint...
Network optimization to minimize Scope 3 emissions, new supplier scorecards, and...
We are back again with the Logility Supply Chain Pulse Newsletter. We hope you f...
Predictable financials are as much, if not more, important today as they were 35...
10 Steps to Sustainable Supply Chain Management A plan for achieving truly...
How can you use AI to better understand how causal forecasting can help your bus...
The urgent need for supply chain resiliency is increasing interest in onsho...
The latest Gartner digital transformation guide for supply chain companies is av...
“If you can’t explain it simply, you don’t understand it…” Albert Eins...
Companies with more agile supply chain practices had service levels that were 7%...
Inventory strategies begin with an efficient network design to effectively balan...
ATLANTA — May 4, 2023 — Logility, Inc., a leader in prescriptive supply chai...
A Textile Distributor plans a seasonal deployment strategy for their product. Th...
At Logility, we’re committed to staying at the forefront of industry trend...
In many cases, supply chain surprises manifest from poor visibility. Reliance on...
As a manufacturing leader, managing constant disruptions, labor shortages, and t...
The supply chain disruptions keep coming, and 2023 appears to be no exception. W...
The gaming world has show everyone in user interface design a better way and Log...
Anything can happen in your supply chain, from unpredictable demand shifts and c...
Logility executives recognized for helping clients future-proof their supply cha...
Year-over-year lowering expectations for revenue, earnings, capital spending, an...
Supply chain network design can help you gain a competitive advantage in uncerta...
The supply chain disruptions keep coming, and 2023 appears to be no exception. W...
The Science and practice of predictive analytics is well established and rapidly...
Review three ways your customers will be impressed by automated order promising,...
ATLANTA – February 27, 2023 – Logility, Inc., a leader in supply chain plann...
ATLANTA – February 22, 2023 – American Software, Inc. (NASDAQ: AMSWA) today ...
ATLANTA – February 16, 2023 – Logility, Inc., a leader in prescriptive suppl...
For decades, engineer-focused software has dominated the supply chain network de...
A key factor in the success of Logility’s network optimization solution is the...
Supply chain issues have put many companies into crisis mode. Avert crises and m...
Solve for demand planning confidence, despite economic uncertainty. Lurkin...
ATLANTA – February 2, 2023 – American Software, Inc. (NASDAQ: AMSWA), a lead...
Measuring the Value of Optimized Inventory in Service Parts The spare parts mark...
With supply chain disruptions on the rise, complexity and uncertainty continue t...
ATLANTA – January 26, 2023 – American Software, Inc. (NASDAQ: AMSWA) has det...
The process manufacturing industry faces unique and daunting supply chain challe...
Key Takeaways Five crucial actions to improve your supply chain financial ...
There is growing pressure for supply chain leaders to find faster, smarter ways ...
There’s been no let-up in speculation over an imminent global recession since ...
As we navigate through the end of the year and the holiday season, we’re also ...
This award honors top software and technology providers that ensure a safe, effi...
Self-tanning leader leverages Logility® Digital Supply Chain Platform to stream...
Gone are the days when supply chain managers could have a rudimentary plan for d...
A Critical Capabilities document is a comparative analysis that scores competing...
Logility powers growth for leader in electrical and digital building infrastruct...
Two consecutive quarters of negative GDP growth is often quoted as sufficient ev...
Deploying products quickly and efficiently is tough when you’re dealing with i...
ATLANTA – November 10, 2022 – Logility, Inc., a leader in supply chain innov...
It’s time to evolve your supply chain from one-size-fits-all to purpose-built....
The traditional formula of minimizing costs and maximizing efficiency is no long...
In a recent survey by McKinsey & Co.1, 80% of respondents said they had increase...
Key Takeaways: At a time of intense supply chain disruption, 57% of organi...
Dwelling on disasters and disruptions gets old sometimes. Yes, we supply chain p...
Make over your supply chain to get visibility into potential future disruptions....
Aims to improve end-to-end supply chain visibility and planning capability by im...
This alliance leverages Wipro’s expertise in business transformation along wit...
Supply chain organizations are like any other part of a business – they’...
The process manufacturing industry faces unique and daunting supply chain challe...
It's incumbent upon businesses today to build the sustainable supply chains that...
According to a recent study by IBM and Celonis on supply chain resiliency, aroun...
Respond quickly, maximize benefits, and identify supply chain opportunities and ...
Logility Congratulates Sherry Boley of ORBIS and Maria Dalmau of Intertape Polym...
While the future of supply chains is uncertain, some pressures have eased. Of co...
Feeling far from bubbly? Get the insights beverage companies need to meet today&...
Why do we build logistics models? This is obviously a rhetorical question. But I...
Global nutritional supplement leader targets greater supply chain transparency w...
In this Q&A, industry expert and Logility EVP and principal Mark Burstein de...
It’s a complex problem, but you can successfully optimize inventory levels wit...
Supply chain disruptions have created extreme uncertainty around supply and dema...
Partnership leverages consulting firm’s deep expertise in retail, grocery, and...
For apparel and soft goods companies, today’s focus is on transparency, speed ...
Solid Start to Fiscal 2023 Highlighted by Continued Strong Growth in Subscriptio...
Competing for the eCommerce supply chain with supply chain modeling software. eC...
According to EY, developing a diverse sourcing strategy is a social and business...
A leader in electrical technologies chooses the Logility® Digital Supply Chain ...
An oversupply of computer chips, new China lockdowns threaten to thwart U.S. eco...
Logility nominated for its work with client iNova Pharmaceuticals ATLANTA, GA (A...
Your ship’s come in! That’s a good thing, right? Much of the merchandi...
It's incumbent upon businesses today to build the sustainable supply chains that...
(Or a cautionary tale about cutting corners.) Are you helping your teenagers wit...
Supply chain design is no longer the “create it, set it, and walk awayR...
Every business ‒ regardless of size, industry, or market ‒ can relate to the...
Today’s supply chains face unprecedented challenges that seem to crop up on a ...
Supply and Demand Chain Executive honors results-proven partnership with large U...
Lack of effective supply chain planning in food & beverage can have life-or-...
Supply chains are becoming more complex. In the search for optimization and auto...
Reach corporate social responsibility goals while improving your economic sustai...
Today’s supply chains face unprecedented challenges that seem to crop up on a ...
Use a two-pronged approach that couples new recruitment approaches with technolo...
With Starboard’s Digital Twin Technology, Logility Clients Can Better Answer ...
Look deeper than ever before. Reduce risk. Increase profits. Make your customers...
While the supply chain talent wars continue, it’s incumbent upon todayR...
A transparent digital supply chain will give you the insights you need for faste...
The fashion industry faces challenges on many fronts: pressure to perform, shift...
The COVID-19 pandemic may have been the catalyst for the ongoing supply chain c...
A global pandemic, changing consumer expectations, and sustainability concerns a...
Continued Strong Growth in Subscription Fees Fuels Revenue Growth of 21% in Q4 a...
Logility chosen for delivering pioneering technology solutions that power today...
Don’t constrain yourself! Real-time insight and the right digital platform...
Interconnected supply chain planning enables faster, more empowered decision-mak...
The COVID-19 pandemic may have been the catalyst for the ongoing supply chain c...
Supply variability today. Where are we at? It’s tempting to think of sup...
ATLANTA, GA – May 10, 2022 – American Software, Inc. (NASDAQ: AMSWA), a lea...
Smart inventory allocation and deployment starts with the right digital supply c...
Don’t ask, “Can we take the order?” Ask, “Should we?” (Is that or...
The pandemic continues to affect supply chains today as digital supply chain man...
U.S.-based supplier of fashion blanks transforms its demand and inventory manage...
If resilience is the panacea to today’s supply chain challenges, it seems that...
This month's newsletter provides the information you need to solve your sourcing...
Letting your vendors own the process and self-manage their corrective action pla...
There are obvious risks involved in sourcing goods from other countries. Managin...
LogDutch apparel licensing company to leverage the Logility ®Digital Supply Cha...
What you don’t know about your suppliers can harm your business and its profit...
It takes cooperation, collaboration, and visibility that enables effective monit...
These best practices will help make supply chain sustainability part of your ope...
This webcast covers the critical elements needed to enable resilience and agilit...
Translate supply and demand variability into powerful inventory strategies.
Logility 21.03 includes new supply network maps and PLM dashboards designed to h...
Many business leaders say they can’t afford sustainability initiatives, bu...
Double-Digit Revenue Growth Driven by Continued Strong Growth in Subscription Fe...
In this month's newsletter, we focus on how to mitigate the escalating challenge...
Allan Dow honored as an influential individual shaping the global food supply ch...
Increasing global regulatory requirements, consumer awareness of business practi...
Today’s marketplace demands businesses invest in supply chain sustainability ...
Value-building sustainable business practices must start at the top — hereR...
There has been a collective sense that 2021 was going to be the pivot, the year ...
ATLANTA – Jan. 27, 2022 – Logility, Inc., a leader in supply chain innovatio...
Building a sustainable fashion supply chain and improving ESG performance will r...
ATLANTA – January 25, 2022 – According to PWC’s December 2021 Global ...
Beginning on January 1, 2023, Germany’s Act on Corporate Due Diligenc...
Supply chain disruptions are commonplace. How do you keep your customers happy w...
ATLANTA, GA (January 13, 2022) – American Software, Inc. (NASDAQ: AMSWA), ...
Planalytics’ weather-driven demand analytics help businesses stay ahead of nev...
Logility recognized for moving the food and beverage supply chain forward effici...
As an addendum to their Software LeaderBoard, RIS has produced the following in-...
We anticipate that the most important supply chain trend this year will be an em...
Enabling technology for sustainable supply chains creates the right level of vis...
Institute supply chain governance fueled by data that only a modern platform can...
Supply Chain Leader Excels in Several Categories of the 2022 RIS Software Leader...
Your customers want to know where their food and drinks have been, not just wher...
Over the years, the fashion industry has championed a movement toward sustainabl...
Manage and maintain corporate social responsibility across your entire network.
Take the next steps in improving supply chain sustainability Key Takeaways...
Bridge the gap between marketing activity and inventory management with a b...
Return to Double-Digit Revenue Growth Driven by Continued Strong Growth in Subsc...
Your ERP system is not the right technology for a sustainable supply chain. Key&...
The unified, digital platform powers better decision-making, scalability, and tr...
Collaboration in life sciences is not new but there is so much more to accomplis...
Increasing scrutiny of labor practices requires supply chain technology that pro...
Gain complete transparency across your supply chain network with traceability.
ATLANTA, GA (November 3, 2021) – American Software, Inc. (NASDAQ: AMSWA), ...
7 ways sustainable supply chains improve your business’s bottom line. Ke...
The cloud is arguably one of the most value-creating technologies of our time. I...
Perhaps it’s little consolation, but the food and beverage (F&B) sector ca...
Technology Leader Gives Students Hands-On Experience With AI-based Supply Chain ...
With the right technology, process manufacturing strategy, planning, and managem...
You need to know what corporate social responsibility is and understand how to l...
9 critical tips for increasing supply chain sustainability in the apparel indust...
Sustainable supply chains aren’t just better for the world; they also gro...
Make better inventory management decisions and gain a deeper understanding of yo...
Many would agree that the life sciences industry has been overlooked in global e...
This eBook provides practical strategies that you can implement now to help you ...
This month we continue the focus on enabling the sustainable supply chain and he...
In our latest release, Logility has doubled down on technology developments to e...
Stricter regulations and changing market conditions mean ensuring supplier susta...
CPG companies can collaborate more effectively, get consensus, and make better d...
Focusing on the evolving business climate, partnership concentrates on embracing...
As the U.S. heads towards its second pandemic winter, there is talk of the far-r...
Supply chain traceability allows you to track the origin and journey o...
Logility 21.02 powers technology to ensure sustainable, ethical practices that s...
What-if analysis for F&B is quickly becoming a tool of choice to coordi...
When it comes to healthcare, one of the greatest hopes people have for themselve...
Customers to benefit from web and mobile technology to help drive value ATLANTA ...
The tools, capabilities, and strategies companies must embrace to achieve t...
Environmental sustainability in the supply chain is top of mind for co...
Supply & Demand Chain Executive Celebrates Influential Women in Supply Chain...
Every industry has a distinct set of challenges, and each supply chain within th...
Life sciences digital supply chain planning will help the industry meet growing ...
ATLANTA – September 13, 2021 – Logility, Inc., a leader in supply chain...
Anybody with a stake at any point along the supply chain will tell you that thin...
Digital Supply Chain Platform provides foundation for industry’s only single p...
Successful products go through a predictable 5-stage life cycle from introductio...
When it comes to supply chain modeling, one size does not fit all, but all of th...
ATLANTA, GA (September 3, 2021) – American Software, Inc. (NASDAQ: AMSWA),...
If the response to COVID-19 taught consumer packaged goods (CPG) companies nothi...
Since the onset of the pandemic, online direct-to-consumer channels have become ...
The voices calling for responsible business practices to extend to the value cha...
Logility named one of SupplyChainBrain’s 100 Great Supply Chain Partners for 1...
Logility customers can now access warehouse and Accelerated Growth in Cloud Serv...
Logility customers can now access warehouse and transportation management soluti...
To ensure long-term growth and protect customer loyalty, businesses need to ...
Here’s how autonomous supply chain planning helps businesses make faster a...
Managing consumer packaged goods inventory is challenging at the best ...
With good reason, we at Logility have been consistently talking and writing abou...
This month we focus on the need for, and benefits of, a sustainable supply chain...
No matter who you are, where you live, or what you do, 2020 was a rough year. Bu...
Gain real-time visibility of customer status and incoming orders, see quantities...
Integrated business planning (IBP) is gaining a lot of traction a...
From shifts in consumer demand to new regulations and disruption in market dynam...
This month we continue to explore the benefits of a dedicated supply chain plann...
Organizational collaboration. It’s no cakewalk – at least, not in the c...
Your business may rely on an enterprise resource planning (ERP) system to fill c...
Agility and speed are on every supply chain executive’s mind. But their well-p...
If you’re interested in hearing how companies are tackling ERP limitations to ...
News headlines may have you believe that supply chains across all categories are...
Berlin Packaging drove increased supply assurance for their customers through Lo...
It’s no longer enough to depend on an enterprise resource planning (ERP) syste...
Panel of Experts to Highlight How Advanced Supply Chain Planning Unites the Exte...
All the signs were there. People just weren’t paying attention. How many...
The Logility® Digital Supply Chain Platform Now Available on Azure Marketplace ...
Food and beverage companies can use demand sensing to predict sudden m...
Partnership Helps Consumer Products, Food and Beverage, and Life Sciences Indust...
Based on thousands of discussions with Logility customers and prospects, we know...
For many supply chain executives, the pandemic leaves behind the legacy of the e...
Record Quarterly Bookings Accelerated Growth in Cloud Services Annual Contract V...
How much time, effort, and estimation goes into figuring out how much product is...
ATLANTA – June 3, 2021 – Logility, Inc., a leader in supply chain innovation...
Tata Technologies will collaborate with Logility to offer digital supply chain t...
Even as the dust begins to settle from one disruption, supply chain organization...
A recent Logility webcast featured industry heavyweights Mark Burstein, Logility...
At the forefront of every change and disruption is an operations function, stret...
Competing in an environment of constant change is always a challenge. But when b...
Businesses today must provide quality products while meeting increasing demands ...
Execution of S&OP through a sales and operations execution process is what s...
A frequent conversation on forecasting best practices among demand planners is t...
Know your vendors and apply consistent policies and procedures. Compliance...
Running a complex, global enterprise from one large suite of seamlessly integrat...
Allan Dow, Anna Palmer, Mark Burstein, Fred Isenberg, and Roger Mayerson Recogni...
ATLANTA – April 27, 2021 – Logility, Inc., a leader in supply chain innova...
Integrated business planning (IBP) is gaining traction as more compani...
Solve manufacturing disruptions with a digital representation of your production...
Unraveling from the complexity of supplier disruption, unexpected consumer behav...
The e-commerce boom has forced virtually every industry to rethink its customer ...
What is integrated business planning (IBP)? Is it identical to sales and operati...
Address operations needs by managing production scheduling complexities using a ...
Carry minimal inventory while ensuring product availability and quick responses ...
Realize the full value of Sales and Operations Planning (S&OP) through the align...
The supply chain is often the first place businesses focus on when looking to qu...
How do you learn from the pandemic to build a better supply chain? What is a...
The power of integrated business planning (IBP) comes in helping companies a...
When a crisis hits, having the right advanced analytics to react to su...
Part Two of Live Series Uncovers Additional Supply Chain Resilience Insights ATL...
Descriptive, predictive and prescriptive analytics should be combined to optimiz...
Businesses today are facing increasing pressure to maintain inventory levels eff...
Traceability will be key in meeting consumer demands for sustainability. The sho...
ATLANTA – February 26, 2021 – Logility, Inc., the leader in supply chain inn...
How do you learn from the pandemic to build a better supply chain?
Subscription Fees Increased 29%, Cloud Services Annual Contract Value Increased ...
Logility is working with a leader in the distribution and logistics space&n...
Two-Part Online Series Explores the Keys to Supply Chain Resilience ATLANTA – ...
This post has been prompted by conversations I’ve had recently&...
Companies that thrive during disruptions have commonalities: they have access to...
One disruption inevitably gives way to another. Can your business thrive versus ...
Supply chain executives worldwide face an ongoing dilemma: improve customer serv...
Customer satisfaction is the driving force behind your supply chain planning and...
You’re committed to rolling out an analytics platform across your supply chain...
Benchmark Based on Global Survey Highlights Logility’s Leadership in Ease of U...
Live Event Explores How to Improve Profitability, Increase Customer Satisfaction...
Building robust supply chain analytics capabilities is more important than ever....
How can manufacturers manage disruption and improve productivity? By using advan...
Change is the only constant in supply chain and often can cloud our visibility. ...
Vendor Compliance is the most critical component to assessing the financial, str...
Live Event Explores Resilient Planning to Better Optimize the Supply Chain of th...
New innovations in supply chain technology are bringing opportunities for needed...
Blockchain Allows Brand Owners and Retailers to Trace Source Materials Ahead of ...
I grew up in a time before people knew much about being eco-friendly or “Green...
An Imperative in Today’s Rapidly Changing World In almost every supply chain p...
Subscription Fees Increased 27%, Cloud Services Annual Contract Value Increased ...
Causal forecasting shines a light on, and isolates, actual demand signals from m...
The ultimate goal of a supply chain planning practitioners is often to optimize ...
Logility Customer Tackles Supply Chain Complexity with Inventory Optimization AT...
When asking the question, “How does machine learning improve supply chain ...
The vast amount of information vying for our attention can make your head spin. ...
Among other aspects of a modern-day business, forecasting has become more comple...
Supply Chain Professionals Recognize Logility for Providing Transformative Suppl...
Supply & Demand Chain Executive Celebrates Influential Women in Supply Chain...
Supply & Demand Chain Executive Celebrates Influential Women in Supply Chain...
Even under the best circumstances, developing production/sourcing plans three, s...
Ask finance managers to name a necessary evil of their responsibilities and many...
Experts in Manufacturing and Food and Beverage Discuss Their Commitment to Servi...
Your Next Stop, The Twilight Zone! I am a big fan of the original Twilight Zone ...
Logility Customers Recognized As Today’s Most Remarkable Leaders During the Mo...
Are we ready? And if so what’s involved? The adoption of artificial intelligen...
As retail companies enter the traditional “back-to-school” selling season, w...
Key Hires in Research & Development, Sales, and Marketing Help Position Ame...
Subscription Fees Increased 43%, Cloud Services Annual Contract Value Increased ...
A quick scan of the Internet will yield a long list of “the biggest-ever suppl...
Forecasting is an “inexact science” that relies on the data available to you...
The global food and beverage supply chain has been stressed to the breaking poin...
Users Highlight Logility’s Depth of Functionality, Ease of Use and Ability to ...
During my Lean/6-Sigma Black Belt studies, while working as a supply-chain manag...
Supply chains are complex. Today, there are new problems to solve, markets are v...
Capabilities Available Soon According to a recent International Data Corporation...
ATLANTA – July 21, 2020 – Logility, Inc., automating the digital transformat...
Quickly Compare Multiple Price and Promotion Scenarios to Determine Most Profita...
Capabilities Available Now The phrase digital transformation is all around us. M...
Prestigious Recognition Highlights How Advanced Supply Chain Analytics Help Body...
Subscription Fees Increased 64%, Cloud Services Annual Contract Value Increased ...
A leading retailer in the home improvement space was having issues delivering ti...
Live Event Explores S&OP and Business Forecasting in the New Normal; Feature...
Deliver the efficiency of top-down planning while respecting individual store di...
Managing the data required for supply chain planning is a bit like raking leaves...
A solid supplier scorecarding program can drive big cost savings in the supply c...
Supply chain analytics user adoption is a challenge that often arises during the...
Annual Ranking Based on Employee Survey of Atlanta-Area Employees ATLANTA – Ma...
Chances are, if you’re in marketing, sales, or one of the more technical aspec...
Unexpected events – pandemics, weather conditions, recessions and more – are...
As a former Director of Supply Chain Management for a multi-national, multi-chan...
Live Event Explores How to Protect Your Organization from Supply Chain Disruptio...
As a process chemical company it is important to optimize and build advanced sup...
Let’s start by busting a myth about Corporate Social Responsibility (CSR): it...
Supply chain transformation involves improving an organization’s abilities to ...
Recently, a colleague recounted a new consulting job he had undertaken a few yea...
In today’s supply chain business environment, improving insights to critical a...
So, the promise of using statistical algorithms, forecasting and predictive anal...
ATLANTA – April 23, 2020 – Logility, Inc., automating the digital transform...
ATLANTA – April 22, 2020 – Logility, Inc., automating the digital transforma...
In the world of commerce, every business ecosystem has a type of supply chain th...
Does Your Company Love Its Sales Forecasting Process? Does your company love its...
Logility is closely monitoring the Coronavirus (COVID-19) situation around the w...
Allan Dow, Karin Bursa, Mark Balte and Henry Canitz Recognized for their Influen...
Supply chain disruptions, like the one we are currently experiencing due to the ...
Supply chain disruptions happen – whether it’s the current COVID-19 situatio...
ATLANTA – April 7, 2020 – Logility, Inc., automating the digital transforma...
Plan for a resilient enterprise with Logility’s AI-based platform This quick v...
Supplier scorecards are globally accepted as a key tool for managing a more prof...
Online Training Catalog Helps Logility Customers Sharpen Supply Chain Skills for...
A consequence of long periods of economic growth, like the one we just had from ...
The promise of demand sensing is that we can do a better job of anticipating and...
In this on-demand webcast, Husqvarna Group, a leading global producer of outdoor...
Work with your Supply Chain Planning Digital Twin Everyone is working hard to fi...
Founded in 1955, Aaron’s is a leader in the lease ownership of name brand furn...
Here’s your two-minute guide to understanding and selecting the right descript...
Live Event Reveals How a Unified Digital Platform Accelerates Decision-Making, I...
Machine learning for demand forecasting has matured to a level of accuracy, tran...
The supply chain professional of the future will need the types of skills you wo...
Over my 25+ year supply chain career I have worked for several distribution-inte...
Supply chains are moving faster and faster and the complexity of data needed to ...
Subscription Fees Increased 57%, Cloud Services Annual Contract Value Increased ...
It’s a great time to pick a hot industry topic and subject it to a “state of...
The accuracy of conventional time-series forecasts eventually hits a ceiling. Wh...
Bring Precision to your Forecasting Multivariate Demand Signal Management isola...
Supply chain organizations are still at the early stages of making the most of a...
Logility Recognized for Helping Food and Beverage Companies Achieve Digital Supp...
ATLANTA – January 21, 2020 – Logility, Inc., automating the digital transfo...
Year after year, survey after survey, forecast accuracy continues to be one of t...
Digital Planning Innovations Sense and Respond to Market Conditions, Accelerate ...
Ladies Fashion Retailer Makes Better Decisions Faster NEW YORK – January 13, 2...
Logility Merges Art and Science of Retail Planning to Remove Risk and Accelerate...
Logility Employees Donate and Volunteer at Santa’s Village to Help Create a Jo...
Logility Named a Leader in 15 Categories of the 2020 RIS Software LeaderBoard AT...
This In The Know video from SupplyChainBrain highlights the perspective of sever...
New Digital Supply Chain Video The supply chain systems of the past are not enou...
Today’s life sciences supply chain is constantly changing as complex market fo...
One of the key challenges facing organizations today is the supply chain talent ...
One of the key challenges facing organizations today is the digital supply chain...
Subscription Fees Increase 64% for the Quarter and Cloud Services Annual Contrac...
Logility Hosts Expert Panel to Share Top Strategies to Attract and Retain Top Su...
Many companies have high hopes when they set out to establish a Lean operating e...
Multi-Echelon Inventory Optimization (MEIO) Shown to Reduce Inventory Investment...
The next episode in the Supply Chain Wars saga, Episode V – The Corporatio...
Artificial Intelligence and Machine Learning Help Transform Data to Make Better ...
Forecasting customer demand has been a critical aspect of business since ancient...
ATLANTA – October 15, 2019 – Logility, Inc., accelerating digital tra...
ATLANTA – October 8, 2019 – Logility, Inc., accelerating digital transforma...
Supply Chain Practitioners Recognize Logility for Powering Digital Supply Chain ...
Every supply chain team must optimize inventory investments with service level p...
ATLANTA – September 10, 2019 – Logility, Inc., a leading provider of collab...
Subscription Fees Increase 41% for the Quarter and Cloud Services Annual Contrac...
Hear how Tillamook hit its growth goals by transforming its team and technology ...
Retailing is currently undergoing one of the most radical seismic shifts with fu...
The path toward Unified Commerce requires both an agile retailing and merchandis...
Retailing is currently undergoing one of its most seismic shifts with further ch...
Tillamook County Creamery Association Transforms Supply Chain to Enhance Custome...
Supply chain is a dynamic field that demands top talent across a wide breadth of...
Evaluating demand forecasting analytics solutions can be a confusing and complex...
Allan Dow, Karin Bursa, Josh Stephens and Henry Canitz Recognized for their Role...
ATLANTA – July 11, 2019 – Logility, Inc., a leading provider of collaborati...
Clarios, formerly Johnson Controls Power Solutions, is the world leader in smart...
Clarios, formerly Johnson Controls Power Solutions, is the world leader in smart...
Tillamook County Creamery Association manufactures and sells high-quality dairy ...
Hear how Finish Line uses Logility’s retail planning optimization solution...
Learn how retailers like Hunkemöller keep pace in today’s unified commerce en...
Excelling at strategic supply chain management, including planning and optimizat...
To establish a firm foundation for success, food and beverage companies must imp...
Live Event Features Hunkemöller, Logility and Columbus Consulting to Uncover ho...
Subscription Fees Increase 47% for the Quarter and Cloud Services Annual Contrac...
Logility Customer Shares How a Digital Platform Helps Support Growth and Product...
Machine learning is a type of supervised or unsupervised artificial intelligence...
Logility Recognized with the Highest Overall Position for Ability to Execute in ...
Logility Customers Share Experiences to Speed Time to Market while Balancing Ser...
Today’s supply chains move at a ferocious pace fueled by multiple data streams...
Innovator in Hose Couplings Turns to Logility to Enhance Visibility and Foster C...
Fifteen years ago, I worked for a company that embraced Lean 6 Sigma as a core o...
I Love You, You’re Perfect, Now Change If you’ve seen the off-Broadw...
Follett Corporation, Siemens Healthineers, Leupold & Stevens, Jockey Interna...
Award Honors Dairy Brand’s Supply Chain Transformation Initiative to Support S...
New Evergreen Upgrade Service Accelerates Supply Chain Innovation ATLANTA – Ma...
Artificial Intelligence-based Solution Automatically Senses, Analyzes and Respon...
Logility Voyager Solutions Transforms Data into Action, Accelerating the Product...
ATLANTA – March 12, 2019 – Logility Inc., a leading provider of collaborati...
Industry Thought Leaders from more than 35 Organizations Including Featured Spea...
Subscription Fees Increase 43% for the Quarter and Cloud Services Annual Contrac...
ATLANTA – February 20, 2019 – Logility, Inc., a leading provider of collabo...
Most companies recognize the importance to improve forecast accuracy and have a ...
Logility Voyager Solutions Honored for Helping Food and Beverage Companies Turn ...
This webcast showcases how CITIZEN WATCH AMERICA uses Logility’s digital s...
You have the place and you have the merchandise. But, do you have your merchandi...
Life sciences supply chain challenges include demand and supply uncertainty. Mar...
ATLANTA – January 24, 2019 – Logility, Inc., a leading provider of collabora...
Apparel is an exciting and rapidly changing industry. Planners need to contend w...
Logility Voyager Solutions to Provide Foundation for Cosmetics Retailer to Bridg...
Retailers are under tremendous pressure to be more agile and responsive, which r...
Retail Industry Survey Names Logility as a Leader in Multiple Customer Satisfact...
Innovations in Machine Learning and Artificial Intelligence Help Tap into Multip...
Logility Voyager Solutions Expands Visibility and Accelerates Planning Cycles fr...
Logility Voyager Solutions Unveils Pinterest-like Functionality to Help Retailer...
Logility Volunteers Staff Santa’s Village to Help Provide a Brighter Holiday S...
Merchandise planning matters. And not just to get the right product in front of ...
Early adopters of AI strategy have enjoyed profit margins greater than 5%. Today...
ATLANTA – December 12, 2018 – Logility, Inc., a leading provider of collabor...
Ok, I will admit it, I am a Star Wars fanatic. I have seen all the movies at lea...
Back in the mid 1990’s when I first entered the Supply Chain field as a freshl...
Subscription Fees Increase 64% for the Quarter and Cloud Services Annual Contrac...
The art of retail is to delight and serve a specific consumer demographic. Manag...
Iconic New Zealand Beverage Company Drawn to Halo’s Industry-Specific Function...
Social media sites like Twitter, Facebook, Google+, LinkedIn and YouTube dominat...
Momentum Increases as Executives Tackle Increasing Data Volumes and the Need to ...
I have an affinity to rock & roll music released from the late 70s through t...
Many consumer package goods (CPG) companies face the same challenges as fashion ...
Session to Discuss the Impact of Artificial Intelligence, Internet of Things and...
BRP and Logility to Discuss Findings from Recent Merchandise Planning Market Stu...
Industry Experts Highlight the Critical Role of Integrated Planning to Drive Vis...
Take the First Step to a Product Concept to Customer Availability Strategy In th...
Cloud Services Annual Contract Value Increases 71% ATLANTA (September 4, 2018) A...
Durable goods, also referred to as hard goods, are items that are not supposed t...
Supply Chain Practitioners Recognize Logility for Driving Noteworthy Supply Chai...
ATLANTA — August 28, 2018 — Logility, Inc., a leading provider of co...
The variety of products available through a growing number of distribution chann...
The consumer durables industry is experiencing significant supply chain threats,...
“The Keys to Creating and Leveraging Actionable Information,” published by P...
Retail demand planning in the past has allowed companies to experience great suc...
Prestigious Recognition Highlights Glen Ravens Success with Logility Voyager S...
ATLANTA — July 31, 2018 — Logility, Inc., a leading provider of coll...
The vast amount of information vying for our attention can make your head spin. ...
Myopia, more commonly referred to as nearsightedness, is a medical condition in ...
How S&OP Drives Supply Chain Optimization Take 60 seconds, okay, maybe 90, ...
Viewing a chemical manufacturing plant from a distance, everything seems so peac...
The ability to analyze performance and determine the root-cause of issues has be...
Cloud Services Annual Contract Value Increases 108%, and Total Revenues Increase...
ATLANTA — June 18, 2018 — Logility, Inc., a leading provider of coll...
The ability to rapidly evaluate multiple scenarios and drive smart business deci...
ATLANTA — June 13, 2018 — Logility, Inc., a leading provider of coll...
ATLANTA — June 12, 2018 — Logility, Inc., a leading provider of coll...
Manufacturers today face a long list of tough supply chain challenges. Supply ch...
Wholesale distributors sit in a precarious location when it comes to supply chai...
Download this addition to the popular eBook, Practical Tips to Improve Demand P...
ATLANTA — June 5, 2018 — Logility, Inc., a leading provider of colla...
As the seasons and styles change, many retailers are faced with a dilemma: what ...
SYDNEY AND ATLANTA — 15 May 2018 — Logility, Inc., a leading provider of col...
Transformation is coming to your supply chain and according to most industry res...
ATLANTA — May 9, 2018 — Logility, Inc., a leading provider of collaborative...
John Glenn became the first American to orbit the Earth on Mercury-Atlas 6 on Fe...
ATLANTA — May 8, 2018 — Logility, Inc., a leading provider of collab...
As someone on the tail end of the Baby Boomer generation, I have a vested intere...
Life sciences companies face a unique set of supply chain demand planning challe...
ATLANTA — April 24, 2018 — Logility Inc., a leading provider of coll...
ATLANTA — April 19, 2018 — Logility, Inc., a leading provider o...
A forecast is more than a projection of future business; it is a request for pro...
ATLANTA — April 17, 2018 — Logility, Inc., a leading provider of collaborat...
Allan Dow, Ed Thompson and Karin Bursa Recognized for Elevating the Role of Supp...
Demand volatility and customer mandates are two of the top three pressures on bu...
In this video, see how merchandise planning, used as part of a comprehensive ret...
Last week we shared five capabilities to help take the Food & Beverage suppl...
This webcast highlights how to drive smart business decisions by harnessing new ...
Food and beverage supply chains face mounting pressure to reduce costs, add more...
Industry Executives Recognize Logility as a Leader in Customer Satisfaction ATLA...
Building robust supply chain analytics capabilities is more important than ever....
Omni-Channel Retailers Take a Consumer-Centric Approach and Transform Disparate ...
ATLANTA — March 6, 2018 — Logility, Inc., a leading provider of collaborativ...
Ashley Furniture Industries, a large global home furnishings manufacturer, opera...
ATLANTA — March 1, 2018 — Logility, Inc., a leading provider of coll...
License Revenues Increase 50%, Cloud Services Annual Contract Value Increases 12...
It seems like the phrase “digital transformation” is everywhere thes...
With increasing frequency, I have noticed blog posts touting the position that &...
Most executives agree that the ability to use demand planning software to genera...
Planning teams face multiple dilemmas including promoting and supporting top lin...
ATLANTA — January 17, 2018 — Logility, Inc., a leading provider...
As a supply chain professional, we need to consider how actions in one part of t...
ATLANTA — January 16, 2018 — Logility, Inc., a leading provider...
Artificial Intelligence and Machine Learning Harness Social Signals to Accelerat...
Network-Wide Multi-Source Distribution to Omni-Channel Locations Based on Need a...
Retail Solutions that Create the Connected Enterprise, Boost Productivity and Dr...
Logility Elves Filled the Stockings of Children in Need for the Tenth Year ATLAN...
The sheer number of options available in consumer electronics products can be ov...
Cloud Services Annual Contract Value Increases 123% for the Quarter ATLANTA (Nov...
ATLANTA — November 28, 2017 — Logility, Inc., a leading provider of collabor...
Lack of visibility across the supply chain can stop even the most innovative omn...
Rapid Insights Driven by Artificial Intelligence and Machine Learning Turn Data ...
Award-winning Software Solution Tracks, Plans & Optimizes Supply Chain Plann...
I recently moved to Arizona and traded my lawnmower and snow-shovels for a salt-...
Ashley Furniture is the third largest home furniture manufacturing company provi...
Travelling often, I run into a frustrating, yet common, problem–delays due...
ATLANTA — October 18, 2017 — Logility, Inc., a leading provider of c...
In an attempt to stretch the shopping budget, many consumers have tried private ...
ATLANTA — October 17, 2017 — Logility, Inc., a leading provider of c...
ATLANTA — October 12, 2017 — Logility, Inc., a leading provider of c...
Logility Voyager Solutions to Help Women's Fashion Retailer Position Invent...
SYDNEY and ATLANTA — 5 October 2017 — Logility, Inc., a leading...
Stop for a moment and imagine the supply chain differently. These were Supply Ch...
Logility Voyager Solutions Recognized for Helping Drive Supply Chain Excellence ...
For more than a decade, I have implemented inventory optimization solutions for ...
Learn how ChemPoint, a leader in the marketing, sales and distribution of consum...
The Consumer Products Industry experienced a 32% growth in active items in 2015 ...
Four Sessions Focus on Integrated Business Planning, Multi–Echelon Inventory O...
Logility Voyager Solutions Recognized for the Twelfth Year for Reliability and C...
You can make your S&OP process pay off for your company — here’s...
ATLANTA — September 7, 2017 — Logility, Inc., a leading provider of collabor...
This year’s Supply Chain Insights’ annual global conference (Live St...
Even under the best circumstances, developing production/sourcing plans three, s...
Cloud Services Annual Contract Value Increases 92% for the Quarter ATLANTA (Augu...
As a classical and jazz musician, I understand the need for both structured and ...
Consumer products companies must balance efficient operations while finding new ...
Over the last 100 years the average lifespan has doubled from 35 years to more t...
Temperatures on the rise; flowers and trees in bloom; and that time of year when...
Logility Voyager SolutionsTM Recognized for Enabling Supply Chain Excellence ATL...
ATLANTA — July 25, 2017 — Logility, Inc., a leading provider of...
Caught Between a Rock and a Hard Place Supply chain leaders often find themselve...
Holidays are complex. Take for example the upcoming Fourth of July holiday in th...
Cloud Services Annual Contract Value Increases 59% for the Quarter ATLANTA (June...
What It Is, Why We Have It, and Some Simple Ideas to Fill the Gap Rising Demand ...
When I hear the term “Lever” my mechanical engineering side comes ou...
ATLANTA — June 6, 2017 — Logility, Inc., a leading provider of colla...
ATLANTA and SYDNEY — 23 May 2017 — Logility, Inc., a leading provide...
Every supply chain team must balance inventory investment with service level per...
ATLANTA — May 17, 2017 — Logility, Inc., a leading provider of colla...
ATLANTA — May 16, 2017 — Logility, Inc., a leading provider of collaborative...
ATLANTA — May 10, 2017 — Logility, Inc., a leading provider of colla...
ATLANTA — May 9, 2017 — Logility, Inc., a leading provider of collaborative ...
Allan Dow, Don Thomas and Mark Balte Honored for Driving Supply Chain Excellence...
Have you ever had something break only to realize the fix is an obscure part? Re...
ChemPoint, Glen Raven Custom Fabrics, LaCrosse Footwear and Sensient Colors Reco...
Is 100% forecast accuracy attainable? Should it be? Wouldn’t that be calle...
Consumer Goods Technology Magazine Names Logility a 2017 Readers Choice Recipi...
Outdoor Sports and Recreation Company Deploys SaaS–based Supply Chain Solution...
I am constantly amazed that an automotive manufacturer will design and market ve...
I am an avid cyclist and recently I was able to secure a great deal on a trike f...
ATLANTA — (March 3, 2017) — American Software, Inc. (AMSWA) announce...
Logility Voyager Solutions Recognized for Helping Food and Beverage Companies Ov...
ATLANTA (February 23, 2017) American Software, Inc. (NASDAQ: AMSWA) today report...
Leading Global Manufacturer Set to Boost Supply Chain Performance with Logility...
Experts from Around the World Join the Lineup to Share Insights and Supply Chain...
ATLANTA — February 15, 2017 — Logility, Inc., a leading provider of advanced...
This is the fifth post in a series where we investigate some of the myths and mi...
This is the fourth in a series of posts that investigates some of the myths or m...
Logility Voyager Solutions Enables Footwear Manufacturer and Retailers Supply ...
This third in a series of posts that investigates some of the S&OP myths and...
The late consultant, educator, and author, Peter Drucker, is often quoted as say...
In this second post in our series of S&OP myths we highlight the need for fl...
ATLANTA — January 24, 2017 — Logility, Inc., a leading provider of collabora...
This is the first of a series of five posts that investigates common myths and m...
RIS News Ranks Logility as an Elite Leader in Customer Satisfaction ATLANTA — ...
Omni–Channel Retailer Achieves Improved Forecast Accuracy and Better Custo...
Research Finds Integrated Planning Pivotal to Omni–Channel Success ATLANTA —...
ATLANTA — January 11, 2017 — Logility, Inc., a leading provider of collabora...
Supply Chain Teams from Around the World Are Set to Discuss Winning Strategies f...
Logility Elves Staffed Santas Village to Give the Gift of the Holidays to Fami...
For retailers, shifting the business to support the new consumer requires adopti...
Over the last few years I have heard a reoccurring theme from many supply chain ...
Global Food and Beverage Color Manufacturer Achieves Greater Forecast Accuracy a...
ATLANTA (December 1, 2016) American Software, Inc. (NASDAQ: AMSWA) today reporte...
Climbing the Ladder of Analytics Value Amid the general buzz in the supply chain...
Does your team face the challenge of having too many options in the supply chain...
Positioning Based on Completeness of Vision and Ability to Execute ATLANTA &mdas...
Today, Lean is a concept, a philosophy, a practice, and a set of tools all wrapp...
Cloud–based Solution Unites Tactical S&OP with Long–range Strategic Plan...
Are we ready? And if so what’s involved? The evolution to using artificial...
Many companies have high hopes when they set out to implement a Lean improvement...
Ferguson, LOreal, Red Wing Shoe Company, and SnapAV Highlight Supply Chain Exc...
ATLANTA — September 15, 2016 — Logility, Inc., a leading provider of collabo...
ATLANTA — September 13, 2016 — Logility Inc., a leading provider of collabor...
Integrated Business Planning (IBP) distills complex, cumbersome and disconnected...
How do you know if meaningful inventory optimization is possible for your life s...
ATLANTA (August 25, 2016) American Software, Inc. (NASDAQ: AMSWA) today reported...
Conundrum: 1) a riddle whose answer is or involves a pun; 2) an intricate and di...
Proven Solution Simplifies and Accelerates Supply Chain and ERP Integration to D...
Process manufacturers face a highly complex supply chain challenge. Starting wit...
Hundreds of companies struggling to improve their supply chain performance have ...
Logility Customer Shares its Supply Chain Transformation Success ATLANTA — Aug...
With so much focus across industry publications, analysts and conference session...
Award Recognizes Modells Sporting Goods Success with Logility Voyager Soluti...
Speakers from PANDORA, ghd, CooperVision, and BESTSELLER Headline the Agenda ATL...
Logility Voyager Solutions Recognized for the Eleventh Year for Driving Supply C...
License Revenues Increase 30%, Cloud Services Annual Contract Value Increases 37...
Supply Chain Transformation Project Leads to 300 Percent Improvement in Forecast...
Logility Voyager Solutions Helps Outdoor Sports and Recreation Company Transform...
With complex supply chains, SKU proliferation and demanding service levels, life...
Hear Groupe Dynamite, a Montreal-based fashion retailer of the popular Dynamite ...
Logility Voyager Solutions to Automate Supply Chain Processes at Fast–Growing ...
Of all factors that affect business success over the long term, most executives ...
ATLANTA — April 21, 2016 — Logility, Inc., a leading provider of col...
Robust Functionality and Usability Distinguish Logility ATLANTA — April 19, 20...
Local Offices Open in Dusseldorf, Malmo, Sao Paulo, and Sydney ATLANTA — A...
Hundreds of companies struggling to improve their supply chain performance have ...
Hear SnapAV, a revolutionary, technology-enabled B2B supplier of intuitively des...
Red Wing Shoe Company is a leading purpose-built footwear company specializing i...
ATLANTA — April 4, 2016 — Logility, Inc., a leading provider of collaborativ...
ATLANTA — March 28, 2016 — Logility, Inc., a leading provider of col...
ATLANTA — March 22, 2016 — Logility, Inc., a leading provider of col...
License Fee Revenues Increase 16%, Total Revenues Increase 5% and Operating Earn...
I became very familiar with risk management early in my professional career. I w...
ATLANTA — February 23, 2016 — Logility, Inc., a leading provider of ...
Logility Voyager Solutions Highlighted for Enabling Supply Chain Excellence at F...
Hear J. R. Simplot, one of the world’s leading agribusiness companies, des...
ATLANTA — January 18, 2016 — At the National Retail Federation'...
ATLANTA — January 18, 2016 — Logility, Inc., a leading provider of advanced ...
The first step toward successful inventory optimization is getting your internal...
Live Webcast — Delivering a Differentiated Supply Chain through Foundational E...
At the core of every retailer’s omni-channel supply chain is the need to b...
Retail Industry Survey Ranks Logility Number One in Overall Performance, ROI, an...
How well can you define the needs, attributes and priorities for each channel an...
License Fee Revenues Increase 85%, Total Revenue Increases 18% and Operating Ear...
Fast-Fashion Retailer Shares its Supply Chain Success with Logility ATLANTA — ...
Supply Chain 2020 will continue collapsing cycles, challenging Supply Chain prof...
How do you handle stores with space constraints? As a retailer I recently spoke ...
ATLANTA — November 5, 2015 — Logility, Inc., a leading provider of c...
ATLANTA — November 9, 2015 — Logility, Inc., a leading provider of c...
Modells Sporting Goods, Moen, Red Wing Shoe Company, Regal Beloit, and The Tim...
Logility and Aberdeen Group Explore Key Factors to Drive Sales and Operations Pl...
Fast–Fashion Global Retailer Transforms its Supply Chain; Stays Ahead of Chang...
Retail References Deliver Exceptionally High Scores for Logility’s Custome...
Regarding supply chain trade-off curves: trade-off, shmade-off, if you don’...
Based on Proven Logility Optimization Engines, Next Generation Solution Delivers...
Rapid Access to Supply Chain Insights across Android and iOS Devices ATLANTA &md...
Supply Chain Industry Experts and Leaders from Simplot and Gartner Step Up to th...
Revenue Increases 16%, Operating Earnings Increases 77%, and Net Earnings Increa...
In Part 1: An S&OP Q&A with SCM Worlds Kevin OMarah we discussed the...
H. Allan Dow Promoted to President and Geoffrey W. Cobleigh Promoted to Senior V...
Recently I had the pleasure to sit down with Kevin O’Marah, Chief Content ...
Recognition Highlights PartyLites Success with Logility Voyager Solutions ATLA...
Customer–led Award Recognizes Outstanding Supply Chain Solutions and Customer ...
Revenue Increases 6%, EBITDA Increases 26%, and Cloud Services Annual Contract V...
ATLANTA — June 17, 2015 — Logility, Inc., a leading provider of coll...
ATLANTA — May 27, 2015 — Logility, Inc., a leading provider of colla...
ATLANTA — May 11, 2015 — Logility, Inc., a leading provider of collaborative...
The other day I was wrapped in a conversation about speed and it reminded me of ...
Allocation processes and their supporting technology are getting a fresh look by...
Global Footwear and Apparel Company Recognized for its Innovative Sales and Oper...
ATLANTA — April 27, 2015 — American Software, Inc. (NASDAQ: AMSWA), a leadin...
ATLANTA — April 21, 2015 — Logility, Inc., a leading provider of collaborati...
Annual Ranking Based on Employee Survey of Atlanta–Area Employees ATLANTA — ...
Logility and Supply Chain Insights Discuss How to Accelerate Supply Chain Planni...
ATLANTA — March 24, 2015 — Logility, Inc., a leading provider of collaborati...
Logility and Consumer Goods Technology Research Shows Significant Opportunity fo...
Logility Named a Consumer Goods Technology 2015 Readers Choice Recipient for the...
ATLANTA (February 26, 2015) American Software, Inc. (NASDAQ: AMSWA) today report...
ATLANTA — February 25, 2015 — Logility, Inc., a leading provider of collabor...
Advanced Composite Curves Deliver Enhanced Sales and Operations Planning, Multi...
Mining and Construction Equipment Manufacturer Turns to Advanced Supply Chain So...
RIS News Ranks Logility Voyager Solutions Number One in Ease of Maintenance and ...
Supply Chain Success Takes Center Stage September 15 — 17, 2015 in San Diego, ...
Logility Voyager Solutions Highlighted for Increasing Supply Chain Success at Fo...
Latest Version Simplifies and Accelerates Retail Planning, Delivers Advanced Ana...
ATLANTA (December 3, 2014) American Software, Inc. (NASDAQ: AMSWA) today reporte...
Research Finds Best-of-Breed Solutions Offer Significant Opportunity for Retaile...
Logility and Deloitte Reveal How the Use of Powerful Business Process and Techno...
ATLANTA — October 22, 2014 — Logility, Inc., a leading supplier of collabora...
ATLANTA — October 21, 2014 — Logility, Inc., a leading supplier of collabora...
ATLANTA — October 16, 2014 — Logility, Inc., a leading supplier of collabora...
ATLANTA — September 23, 2014 — Logility Inc., a leading supplier of collabor...
Educational Session at CSCMP Global Conference 2014 Highlights Best Practices to...
A unified global supply chain management planning system is a key factor that fa...
License Fee Revenues Increase 36% and Total Revenues Increase 7% for the quarter...
Companies often overlook important nuances as they try to segment their customer...
Award Recognizes Massimo Zanetti Beverage USAs Success with Logility Voyager S...
Merchandise allocation is a key component of retail planning and is crucial for ...
Caribou Coffee and Logility explore Caribou’s best practices in expanding ...
ATLANTA (June 25, 2014) American Software, Inc. (NASDAQ: AMSWA) today reported p...
Live APICS Webcast Discusses Best Practices in Improving Forecast Accuracy, Supp...
Spreadsheet use pervades every aspect of corporate life and supply chain managem...
On May 30, 2014 we announced MID Retail, a provider of retail allocation and mer...
Award–winning Retail Allocation and Merchandise Planning Software Extends Logi...
Inbound Logistics Names Logility to its Annual List for Enabling Logistics Excel...
ATLANTA — May 15, 2014 — Logility Inc., a leading supplier of collaborative ...
Leading HVACR Distributor Recognized for Driving Significant Supply Chain Improv...
American Hotel Register, Caribou Coffee, Gear for Sports, Johnstone Supply, and ...
Exceptional Leadership and Vision Help Transform Global Footwear and Apparel Sup...
Mobile Application Delivers Advanced Supply Chain Functionality across Android, ...
On Demand Simulation and Scenario Analysis, Social Collaboration, and Unified Da...
Architected for the Cloud and Designed for Speed and Agility, Logility Voyager S...
ATLANTA — March 04, 2014 — Logility Inc., a leading supplier of collaborativ...
ATLANTA — March 3, 2014 — Logility Inc., a leading supplier of collaborative...
Operating Earnings Increase 54% for the Quarter ATLANTA (February 27, 2014) Amer...
Deloitte and Logility discuss Retail Supply Chain Collaboration: 3 Catalysts for...
Logility and Deloitte Reveal How Retailers Can Turn Their Supply Chains into a C...
Best–in–Class Companies Turn to Best–of–Breed Supply Chain Solutions to ...
Readers Choice Awards Give Logility Voyager Solutions Top Ranking for Customer S...
Logility Voyager Solutions Enables Food & Beverage Companies to Overcome Com...
Leading HVACR Distributor Drives Increased Visibility and Service Across its 370...
License Fee Revenues Increase 13% and GAAP Net Earnings Increase 33% for the Qua...
Learn How the Right Metrics Can Drive Supply Chain Performance ATLANTA — Novem...
Supply Chain Industry Leaders and Visionaries from Aberdeen Group and Under Armo...
More than Eighty Percent Have a Formal S&OP Process in Place; Yet Seventy–...
Report Highlights Key Strengths in Logility Voyager Solutions Capabilities, Port...
ATLANTA — October 15, 2013 — Logility Inc., a leading supplier of collaborat...
You’ll note in the headline I use the preposition “of” instead...
Footwear and Apparel Manufacturer Discusses How it Decreased Inventory and Impro...
ATLANTA (August 29, 2013) American Software, Inc. (NASDAQ: AMSWA) today reported...
Nominations Highlight Excellence and Reliability as Key Attributes of Logility V...
Leading Footwear Manufacturer Discusses the Journey Away From Spreadsheets to Ad...
The apparel industry uniquely combines the characteristics of make-to-stock manu...
In a business based on time-limited seasonal harvests, such as a food supply cha...
I was asked an interesting question last week which made me think of the nature ...
Choice of Deployment Options Accelerates the Benefits of Supply Chain Initiative...
ATLANTA (June 20, 2013) American Software, Inc. (NASDAQ: AMSWA) today reported p...
Proven Methodology Speeds Integration, Reduces Risk and Drives Quicker ROI ATLAN...
ATLANTA — June 17, 2013 — Logility Inc., a leading supplier of collaborative...
Running an entire enterprise from one large suite of seamlessly integrated appli...
As supply chain teams demand the power of supply chain optimization solutions, t...
Leading Global Manufacturers Recognized for their Supply Chain Innovation ATLANT...
Award Recognizes the Top 100 Most Impactful Supply Chain Projects of the Year AT...
Inbound Logistics Names Logility to its Annual List for Enabling Logistics Excel...
Annual Ranking Based on Employee Survey of Atlanta–Area Employers ATLANTA — ...
Over the last dozen years Inventory Optimization (IO) has assumed a crucial role...
As I talk to customers and practitioners from all corners of the supply chain wo...
Experts Outline Best Practices and Share the Benefits of an MEIO Approach ATLANT...
If you’re reading this, chances are you’ve either lived through a su...
Logility Named a TAG Top 40 Innovative Technology Company ATLANTA — March 19, ...
Live Event Presented by Logility and Deloitte Reveals Sales and Operations Plann...
ATLANTA (February 27, 2013) American Software, Inc. (NASDAQ: AMSWA) today report...
Readers Ranks Logility a Leading Supply Chain Management Vendor ATLANTA — Febr...
ATLANTA — February 20, 2013 — Logility, Inc., a leading supplier of collabor...
From storage capacity options in laptop computers to diameters of PVC pipe, from...
Live APICS Event to Highlight Best Practices in Developing a Successful Collabor...
This sounds a bit like the old tongue twister ‘how much wood could a woodchuck...
Estimating future demand forecast accuracy is crucial for success yet frustratin...
According to Gartner, the typical company provides support services and parts fo...
Estimating future demand is one of the most fundamentally valuable, but frustrat...
Learn how Continental Mills, producer of dry bakery mix products, including Grai...
Recently, we were asked by the management of a manufacturer to help them assess ...
The best recipe for an optimized food manufacturing supply chain involves a blen...
Berry Global’s products are integral to the success of many of the world’s b...
A key characteristic of best-in-class companies is their success in using invent...
In the consumer electronics industry, it is crucial to optimize inventory becaus...
Private label manufacturing is a demanding discipline characterized by high serv...
CooperVision and Logility discuss the challenges of managing a broad portfolio ...
Intertape Polymer Group (IPG) is one of the largest tape and film manufacturers ...
VF Corporation is the world’s largest apparel company with more than $12.4 bil...
Increasingly companies are recognizing the value of aftermarket parts and servic...
Dynamic Sales & Operations Planning (S&OP) can meet the demands of accel...
During an economic recovery, strategic companies leverage supply chain planning ...
Hear why “thinking green” is more than just a trend in today’s...
The keys to competitive advantage are managing supply chain complexity while dri...
Intertape Polymer Group (IPG) improved its demand, inventory and replenishment ...
It’s a fact — using supply chain software to make Inventory Optimization (IO...
In a supply chain management software context, Vendor Managed Inventory (VMI) or...
The branded apparel industry is at a crossroads as new merchandise planning chal...
Inventory optimization software from supply chain management companies can act a...
A robust and successful SCM system that supports Sales and Operations Planning (...
Studies show that a majority of CFOs believe the supply chain and inventory opti...
Inventory Optimization (IO) is a loosely used term in the industry. You’ll see...
Sales and operations planning (S&OP) is a process designed to unite all key ...
With an introduction specific to the Australian supply chain market, this invent...
Chances are, if you’re in marketing, sales, or one of the more technical aspec...
Try adjusting your search or filter to find what you’re looking for.