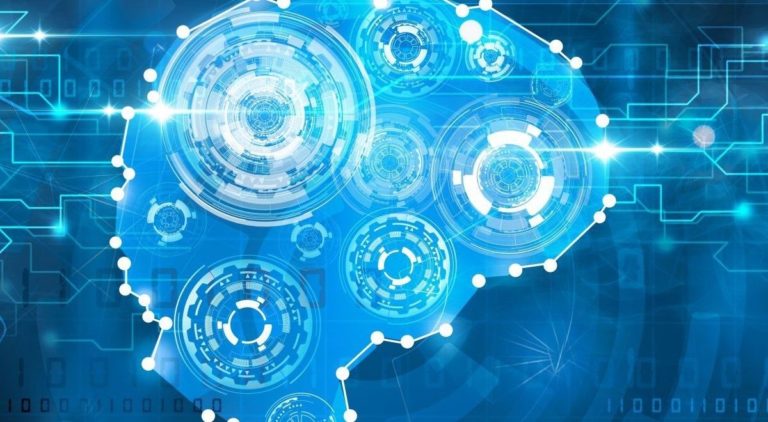
When it comes to designing and creating technology solutions for supply chain analytics, this is not a throw-away idea buried in a long-forgotten PowerPoint presentation. Logility’s point of view is clear: we prefer to start with the user experience. We identify the optimal way for an expert to interact with data to improve decisions. We then work backwards to find the appropriate solutions that enhance the user experience so that the expert has more data at hand for making better decisions. The expert user interacts with data, reports, and forecasts. The variance from forecast identifies business processes that decision makers will want to understand and take management action on. Therefore, always start with a user’s needs and work backwards.
The Influence of New and Emerging Technologies
A quick examination of some recent examples of the influence of what are still considered emerging technologies — such as machine learning and artificial intelligence (AI) — demonstrates that the human element always was, and in fact always must be, paramount. The early hype focused on harnessing the advantages that machines have over humans. Math, speed, brute force, impartiality: this was exhilarating stuff, but it was never about a diminished role for humans.
In 2016, Lowe’s introduced LoweBot, an autonomous retail service robot in Lowe’s stores throughout the San Francisco Bay area. For the customer, LoweBot can find products in multiple languages and effectively navigate the store. As LoweBot helps customers with simple questions, it enables employees to spend more time offering their expertise and specialty knowledge to customers. Furthermore, LoweBot is able to assist with inventory monitoring in real time, which helps employees detect patterns that might guide future business decisions. Employees are receiving a double benefit: more time to focus on the strategic versus the mundane and real-time inputs to improve inventory management. What a fascinating example of how machine learning improves supply chain performance.
Or consider Walmart’s use of satellite photography and drones for scanning its warehouses. These technologies offer speed, accuracy, and attention to detail. They dutifully report what they find but offer no commentary.
In 2015, IBM acquired The Weather Company. According to IBM’s website, businesses can now make better decisions by using weather data. “Our solutions connect newscasters, airline pilots, energy traders, insurance executives, state agency employees, retail management and more to the weather intel they need, on any device. We aggregate the deepest, richest data sets — both business and consumer — to deliver personal, reliable and actionable weather information, analytics and insight.”
The Machine Learning Evolution
Using weather data as context, we’re living through a progression that looks something like this:
- Everyone has known forever that weather impacts the sales of many goods and services. Absent ML technology, humans did the best they could to forecast demand and plan inventory. Mom’s lemonade-stand wisdom held sway: “Make one pitcher at a time while it’s raining.”
- Next was the advent of the descriptive power of big data, enabled by giving powerful computers access to vast troves of digital data on the Internet. Call it the era of correlations. As one Walmart executive put it, “We don’t know why berries sell better when the temperature is below 80 degrees. We just go with it. We stock more berries and sell more berries.”
- The next frontier: efficient machine learning implementations that enable machines to learn, refine and steadily improve the utility offered to decision-makers.
The Next Frontier
What does that next frontier look like in practical terms? To start with, it means closing the loop by benchmarking results, providing feedback and refining plans, again and again. Ultimately, machine learning becomes a closed system when the data flows are highly automated, modeling is continuously updated based on the latest information, and the output of the data processing is an automated input to a business decision — like a forecasting decision or an inventory position decision.
And it means that the benefits of automation are always balanced against the understanding that positive business results come from human actions. Machine learning has much to offer the modern-day supply chain, but we pesky humans continue to play the most important role. In simple terms, that ‘most important role’ is the cycle of observation followed by critical thinking followed by action.
Interested in finding out more about machine learning as part of an overall supply chain planning strategy? Take a look at our eBook The Role of Machine Learning in Supply Chain Planning