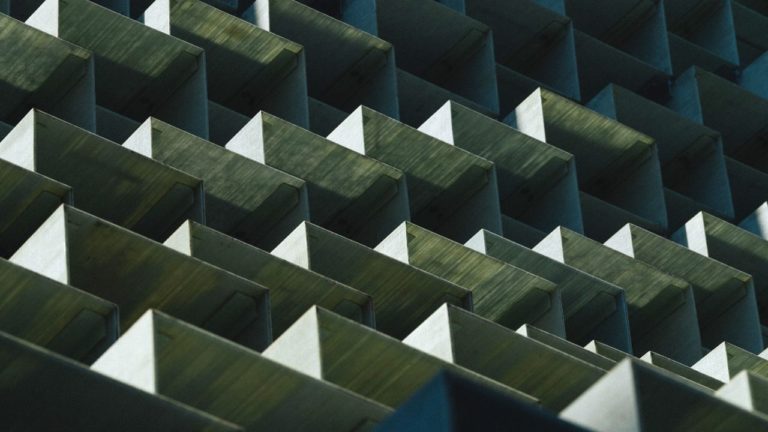
It’s no secret that an improvement in forecast accuracy, especially at the SKU level, can have a significant impact in reducing downstream supply chain costs and improving customer service levels. Accurate forecasts help minimize inventory, maximize production efficiency, streamline purchasing, optimize distribution, maximize customer service, ensure confidence in company financial projections. Gartner, for example, places demand forecasts at the top of their Hierarchy of Supply Chain Metrics to highlight the impact of forecasts throughout the supply chain.
Improving forecast accuracy by just one percent can significantly reduce finished goods inventory, lower transportation costs, decrease inventory obsolescence and increase perfect order performance. Considering the number of benefits attributed to an improved forecast, one could assume that supply chain practitioners would have already perfected or nearly perfected the forecasting process. Sadly, this is far from the truth. On the bright side however, it doesn’t have to be this way.
Time and time again we conduct surveys and find the main roadblocks to achieving forecast accuracy nirvana are the prolific use of spreadsheets and a combination of legacy systems and ERP modules. The lack of modern, digital technology powered by artificial intelligence (AI) is significantly limiting the potential for companies to profitably grow and deliver the service today’s customers expect.
A confluence of advanced forecasting solutions, increased computer processing power, growing availability of Big Data, and a younger more tech-savvy workforce makes the time right to retire these legacy practices to enable more efficient and effective demand optimization capabilities and drive higher forecast accuracy. While not rocket science, drastically improving SKU-level demand forecasts will require investments in people, process, data, and enabling solutions.
The Changing Role of the Planner
Advanced demand planning solutions today are intuitive and easy to use, graphical and packed full of advanced capabilities that analyze historical data and forward looking demand signals. These systems have embedded machine learning, collaborative workflow, and configurable alerts to automate the routine. All of this allows today’s planner to focus on value-added analysis and decisions that further improve forecast accuracy and drive business performance improvements.
Although advanced demand planning solutions can do much of the heavy lifting through automation and artificial intelligence, skilled demand planners remain a necessity to handle exceptions, collaborate with internal and external stakeholders and ensure operations are aligned with executive strategy. However with the implementation of an advanced demand planning solution the demand planner will spend more of their time analyzing data, solving problems and developing value. This change in job responsibilities may require educating existing planners or hiring new resources with more data analytics experience and training.
The Data Opportunity
Data is everywhere and the availability of data that can be used to enhance demand forecasts continues to grow exponentially. This creates a data collection and management problem that many companies are not equipped to handle. Much of this data originates outside of a company’s enterprise systems, and can’t be managed within ERP systems. Furthermore, demand data that does reside within a company’s enterprise systems may be outdated and fraught with errors. Without clean, consistent data, demand planners will struggle to create accurate forecasts. Companies need to invest in supply chain data management capabilities to effectively collect, cleanse and maintain all the additional Big Data that is now available to improve forecast accuracy.
The Rise of AI and Automation
As you can imagine, automation and artificial intelligence driven processes are significantly changing the demand planning process. Looking ahead, much less time and effort will be spent on data crunching and more on value adding analysis and problem solving. Therefore, a significant amount of the effort involved in maturing your demand planning process will involve process reengineering to take full advantage of your enhanced system, additional data sources and planner analysis skills. A focus on life cycle planning and using the right forecast method tuned to maximize forecast accuracy at different phases of a product’s life cycle needs to be developed. (Learn more in: 2020 Planning Tip: Eight Methods to Improve Forecast Accuracy) Life cycle planning is an extremely important part of any mature forecasting process for those companies that introduce and retire products on a regular basis.
Honestly, I am disappointed we haven’t witnessed more improvement in our ability to accurately forecast product demand over the last 25+ years. While proven to be unreliable, error-prone and slow, most companies still rely on spreadsheets for their demand planning needs. Sometimes it is just hard to break a long-standing habit, no matter how bad it is for you and those around you. However, I am optimistic that larger improvements will take place faster over the coming years. Afterall, with the pace of digital business, the complexity of operating in global markets, and the continued scrutiny on how to improve razor thin margins, companies can no longer operate with inaccurate and delayed product forecasts if they hope to survive. So yes it is possible to achieve high SKU-level forecast accuracy. Start by tapping into Logility…