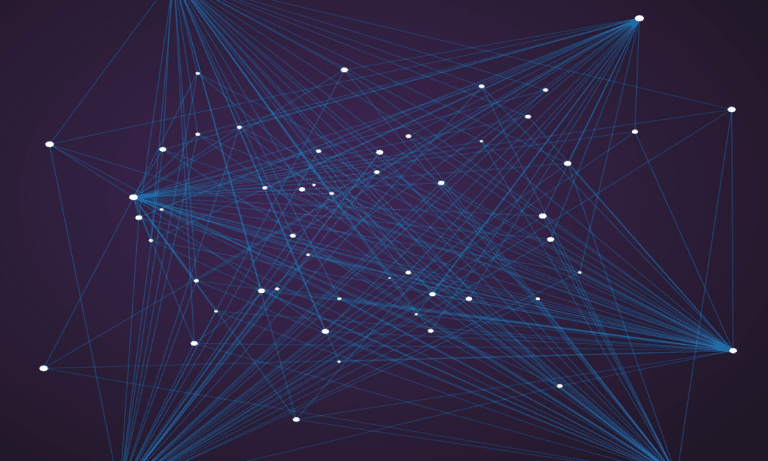
Capabilities Available Now
The ongoing challenges to attract and retain talent, meet rising customer expectations and stay ahead of fierce competition mean companies are missing opportunities for improved margins, reduced working capital investments and greater service levels. The convergence of increased data availability and technology advancements makes now the right time to embrace a ML-powered supply chain. Luckily there are a number of AI/ML capabilities available today to automate supply chain processes and augment your supply chain team’s ability to make decisions. These include:
Optimized Forecast Algorithm Selection capabilities automatically select the best forecast algorithm whenever new data is added to demand history. Unfortunately, many companies tend to use only a few forecasting algorithms across their entire product portfolio. To make matters worse, the assigned algorithms are rarely reviewed and when they are very little consideration is given to a product’s life-cycle.
Commonly referred to as a ‘Best-Fit’ algorithm, this ML-powered capability automatically compares the forecast accuracy of every forecasted item against every available forecast algorithm, ultimately choosing the algorithm that minimizes forecast error. ‘Best-Fit’ forecast algorithm selection ensures that forecast accuracy is optimized throughout a product’s life-cycle.
Forecast Parameter Optimization capabilities continuously sense, analyze and update forecast planning parameters used for trend, seasonality, smoothing, etc. to improve forecast accuracy. For many companies, algorithm parameters at the forecasted item level are rarely reviewed or adjusted due to a lack of manpower or the lack of advanced statistical knowledge. This type of ML-powered capability autonomously analyzes demand signals against actual forecast performance to proactively adjust forecast algorithm parameters to optimize forecast accuracy.
Demand Outlier Adjustment capabilities automatically detect anomalous demand history data points and substitute a corrected value. Significant time and effort can be spent correcting and adjusting data history to account for anomalies due to stock outs, competitors’ promotional programs, unplanned disruptions or non-repeating events. These types of solutions use machine learning to analyze both uncorrected and corrected forecasts to improve forecast accuracy by first ‘learning’ what bad data looks like and then making substitutions for anomalies. An ML-powered demand outlier adjustment solution reduces time spent on data manipulation, freeing up planner time to work on more valuable activities.
Demand Sensing from Unstructured Data capabilities use machine learning pattern recognition and natural language processing to read and analyze ‘Big Data’ to recognize complex patterns and provide data insights. In today’s social media driven economy, consumer likes and wants can shift very quickly. All it takes is a celebrity tweet about a favorite product to spike demand, making it nearly impossible to stay abreast of all the latest social media data. Advanced demand sensing solutions automatically analyze terabytes of unstructured data to determine “sentiment” and quickly predict an impact on short- and longer-term demand.
Probabilistic Demand Simulation capabilities use machine learning to understand forecast variability at the record level. In contrast to single-valued forecasts, probabilistic demand simulation builds a range of possible demand forecasts. Advanced solutions use this understanding of variability to create randomized forecasts which are used in n-tiered, supply constrained ‘Digital Twin’ simulations to predict supply chain resilience. The incorporation of product level revenue and profit information into these Monte Carlo type simulations helps planners assess the risks of meeting both volumetric and financial targets.
Automatic Data Cleansing and Parameter Population capabilities use machine learning to recognize incomplete or inaccurate supply chain data, and either automatically applies the correct data or alerts the appropriate data manager to take corrective action. Poor data quality is a common problem that affects the ability to develop accurate plans. Advanced solutions that automatically cleanse data and populate supply chain parameters ensure timely and accurate data is available for supply chain planning operations.
Machine Learning solutions learn, analyze and adapt as new data is absorbed. ML solutions can understand complex concepts, solve complex problems and make complex decisions. Some forms of AI mimic the human brain, learning and evolving over time. According to Supply Chain Insights, “CEOs expect supply chain leaders to prepare for digital business and want to know how they intend to develop capabilities and use advanced technologies like artificial intelligence to create a flexible, agile and responsive digital supply chain.”3
As a Supply Chain Practitioner, are you prepared to meet your C-Level executives’ digital supply chain expectations?
Look for part 2 of this blog, “Machine Learning (ML) in Supply Chain Planning (Part 2 of 2) – Capabilities Available Soon”