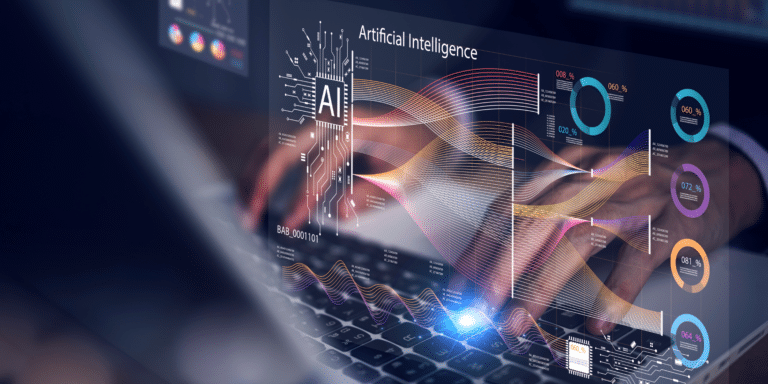
Leveraging AI for Faster, Strategic Decision Making
There is a lot of information out there around generative AI, and it’s difficult to separate fact from fiction. As a member of Logility’s research and development team with a specialization in generative AI, I have a front row seat to witness the rapid expansion of artificial intelligence technology. AI has presented challenges and opportunities for business leaders seeking to leverage its potential across their organizations to improve efficiency and increase profitability. In this blog, I‘ll address 10 common generative AI myths to demonstrate the value of this exciting technology.
Myth 1: Generative AI is a recent development over the last couple of years
Generative AI has risen to the forefront of public awareness in the last year or two. However, AI is based on artificial intelligence and machine learning methodologies that have continuously evolved since the 1950’s. During this time, the same AI tools that underwrite new technologies have been key in improving efficiency and optimizing all areas of logistics and supply chain processes including forecasting, supply planning, inventory management, manufacturing, network optimization, and more.
Myth 2: Generative AI is unable to keep your data private
One of our top concerns is that clients have complete confidence that their data is safe and secure. Generative AI absolutely can be built with measures to safeguard privacy. For example, with Logility GenAI your data is safeguarded with advanced encryption protocols and robust access controls to ensure your sensitive information remains confidential and protected.
Safely Mine Your Supply Chain Data with GenAI
Watch the DemoMyth 3: Generative AI is best as a black box
At first glance, the prospect of generative AI supporting a 100% automated workflow might seem like a desired goal for your supply chain processes. However, experienced day-to-day planners know that human oversight is crucial for good outcomes when determining strategies, developing forecasts, building supply plans, and managing inventory. Smooth integration of generative AI technology with subject matter experts is especially important in cases of exceptions, last minute requests, and unexpected disruptions.
Myth 4: Generative AI is always smarter than humans
Yes, generative AI has strengths beyond human capabilities. It can learn faster than humans and is trained to process and analyze huge amounts of information based on training data, algorithms, and statistical models. However, generative AI can’t extrapolate contextual information from situations or use human concepts of understanding, feelings, and intuition.
For example, suppose an order for a key customer is going to be late. Because of a personal relationship, the supply chain manager knows they can call their colleague from sourcing to lean on their vendors to get the shipments expedited. Generative AI can only act based on what it’s learned from its training data whereas the supply chain manager can use their intuition based on the context of the situation to make decisions and act.
Myth 5: Generative AI will reduce the workforce in your company
Generative AI complements, not replaces, a human workforce by making jobs easier and allowing workers to focus more on strategic decision-making rather than tedious repetitive labor.
Imagine when getting ready for their bi-weekly S&OP meeting, an analyst must determine which products require additional scrutiny along with the most important reports and KPIs. A fine-tuned generative AI assistant will automatically generate this data for the analyst before the meeting, freeing the analyst to focus on interpreting the latest metrics and planning. The analyst responsibilities are now elevated from digging through data to decision making based on key factors.
Myth 6: Bigger is Better
The idea that “bigger is better” when it comes to generative AI models is a common misconception. Without getting too technical here, generative AI models can have billions of parameters, that is, the mathematical weights and biases for the models. For example, Meta’s Llama2 has up to 70 billion parameters, and it is rumored that OpenAI’s G PT-4 has 1.7 trillion parameters. These models are so large in part because they are purported experts in everything. Small models can perform the same or better than these huge models when trained and fine-tuned on a very specific domain. This is because they are focused on deep subject matter instead of the broad range of topics of the bigger models.
Myth 7: Generative AI solutions are 100% reliable and consistent
Even with its amazing capabilities, relying on generative AI predictions alone without human validation can lead to poor outcomes. You may have even heard of “hallucinations”, when a chatbot makes up an answer that is not based on real data. We can head off these kinds of bad outcomes by ensuring transparency of the inputs and approaches used by the generative AI model. Capabilities of GenAI show the user the data source that corresponds to the answer for each question the user asks. This provides users with confidence in the response as well as a chance to identify inaccuracies if they exist.
Myth 8: Generative AI is immune to biases present in training data
Generative AI produces predictions based on its training data. If the training data is “biased”, or an inaccurate representation of reality, then the outcomes will be predicated on these biases.
For example, an inventory manager is under immense pressure to reduce inventory costs. To do this, they override their initial optimized plan and set inventory policies to reduce stock by a small percentage. An AI model could use these biased policies to generate an inventory plan which leads to shortages and lost sales. In this example, the inherent bias in the AI inventory model’s inputs leads to decreased profitability. With the right solution, these issues can be address by interrogating model inputs and assumptions, and training models to be on the lookout and correct for bias.
Myth 9: Generative AI has thoughts and feelings
Generative AI is not sentient. Even though it sometimes seems to be, generative AI doesn’t have feelings or empathy and it doesn’t actually understand what it’s saying in the same way humans understand. When you ask a chatbot a question, the response is a set of words or phrases generated by a complex prediction model. Although responses are often extremely reliable and accurate, they are based on statistically “likely” combinations of words and characters, not any feelings or emotions.
Myth 10: Generative AI can replace human intuition and decision-making
As we’ve discussed above, human intuition is often required for reliable decision-making. Collaboration between generative AI models and human experience gives us the best of both worlds in creating robust solutions in supply chain planning and management.
To wrap up, I hope you’ve been able to gain a little insight into generative AI and cleared up some possible generative AI myths and misconceptions. Logility is focused on integrating these powerful capabilities throughout our platform. We pair technical and subject matter expertise to make sure your business has the tools it needs to answer planning questions and keep business running smoothly, efficiently, and profitably.
AI-First Demand Forecasting
How Human-Machine Collaboration Cuts Costs, Error, and Implementation Time
Free eBookWritten by
Lynne Goldsman
VP, Research & Development
Short bio
Lynne Goldsman works on developing innovative generative AI solutions at Logility. Lynne previously helped lead Logility’s innovation team to research and create state of the art outcomes for clients. Her career spans over 25 years of serving in many roles as research analyst, data scientist, developer, and supply chain consultant.