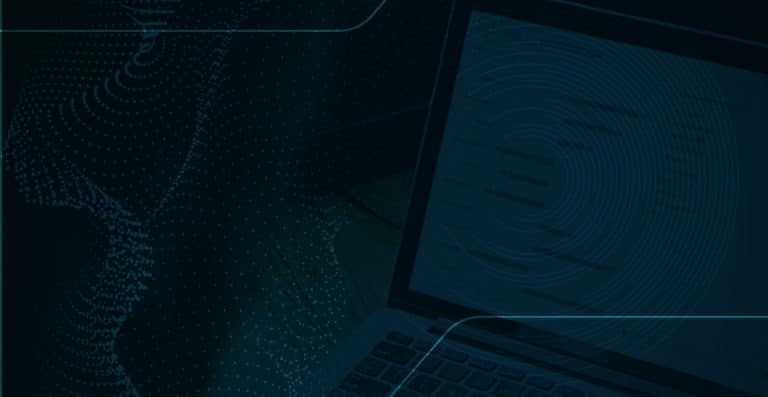
The Science and practice of predictive analytics is well established and rapidly gaining ground in the public and private sectors. It’s no longer considered magic because we now have advanced analytics systems that harness and organize massive amounts of disparate data and model that big data in ways that allow humans to be proactive and make informed decisions.
How would your supply chain decision-making be enhanced if you had the power to harness the data of the past into decisions for the future using predictive analytics modeling?
What are Predictive Analytics for Supply Chain?
Predictive analytics encompasses a variety of statistical techniques from predictive modeling, machine learning, and data mining that analyze current and historical facts to make predictions about future or otherwise unknown events.
Predictive models exploit patterns found in historical and transactional data to identify risks and opportunities. These models capture relationships amongst many factors to allow assessment of risk or potential associated with a set of conditions, guiding decision-making with better accuracy and significant cost savings.
How can big data lead to supply chain optimization?
Let’s examine two popular applications: supply chain optimization and baseball. How can predictive analytics effectively address these seemingly unrelated topics? Because at a macro level the issues are identical. Consider this abbreviated chronology of our quest to make better, faster, data-driven decisions regardless of the setting and the objectives:
- We had no data. We used unstructured observations and gut feel
- We got some data, but it was incomplete and resided in silos
- We got more (and more comprehensive) data, eliminated silos, filled in the gaps, but lacked modeling tools. This was the era of data-rich but information-poor, the big data conundrum.
Today, predictive analytics tools allow us to compare possible outcomes of events using scenario analysis and foresee challenges and potential disruptions before they happen.
Supply Chain Optimization – Use Case for a Domestic Brewery
Our supply chain optimization use case comes from a top-10 domestic brewery that used Logility’s predictive analytics capabilities to gain better insight into production. Before Logility, this brewery had plenty of data, but was unable to make sense of it and “make it tell us something useful about the future”. Sound familiar?
The data needed to be more easily translated into actionable information for managers and executives. The company had a variety of tools in-house, but the fragmented technical environment was too difficult to manage for quick scalability. They needed a powerful, analytics-driven solution to integrate and transform the data from their disparate systems, along with a front end for visual analytics, designed for the specific challenges of the beverage industry.
A key point of differentiation for Logility was the ability to link multiple data sources to a single supply chain planning platform with reporting and analytics capabilities built into the functionality. Logility’s rapid integration framework enables a one-time setup of the platform, followed by easy report creation and access to predictive analytics by business users. An early win included creating a daily shipments and depletions report for the CFO. Using a mobile-ready interface, the CFO can quickly scan variances each morning and immediately drill down to SKU and account-level data to see what’s driving exceptions.
Based on these early victories, the brewery believes that the early detection of production efficiencies will yield up to $800,000 savings within the first 18 months. In addition, the company points to a two FTE reduction (about $300,000 annually), and faster decision-making by business managers.
The Baseball Analogy
Now let’s consider the case of building a winning team in baseball, and the use of sabermetrics. The premise of sabermetrics is that the historically most common measure of performance, the runs-batted-in percentage (RBI), was an incomplete measure of the likelihood that a team would win a championship. Baseball statisticians, now called “sabermetricians”, have figured out how to use all the available data on a player’s performance to make a better decision on which players to combine onto a team. This is a great example of predictive analytics applied to baseball.
You may have seen this portrayed in the movie “Moneyball”, where the proponent of sabermetrics was trained in economics and computer programming, was arguing for the managers of the baseball team to try a different and more scientific approach. The sabermetrician focused on a new metric: On Base Percentage (OBP). The sabermetrician found that getting on base by any means is foundational — you cannot be batted in if you are not on base. Sabermetrics Identified the first link in a chain of causal events that lead to success: focus on getting on base by any means including walks and being hit by the pitcher.
Even more important, the Moneyball sabermetrician knew that decisions on which players to recruit and who to trade were constrained by budget and availability. With other teams pursuing recruits with the highest RBI statistic, the sabermetrician knew there was an arbitrage opportunity — recruit older players with high OBP, even if they were near the end of their careers and being traded away by other teams because their RBI statistic had dropped off.
The dominance of sabermetrics in modern baseball is analogous to how big data and advanced predictive analytics is now coming to dominate modern supply chain optimization. Combining more and more data into a large ecosystem affords a broader analysis space, leading to new insights that would never be found if the data processing were not blended and automated. Identifying conditions that have a larger-than-average value multiplier (arbitrage) is more powerful when the search for arbitrage is informed by big data. And in both baseball and supply chain management, you need financial metrics in order to choose the best strategy.
As you can see, predictive analytics and the underlying tools that support the discipline can be applied in many settings. People like to solve problems, but they need the right information. As business leaders we need to make sure they have it and then set them free.