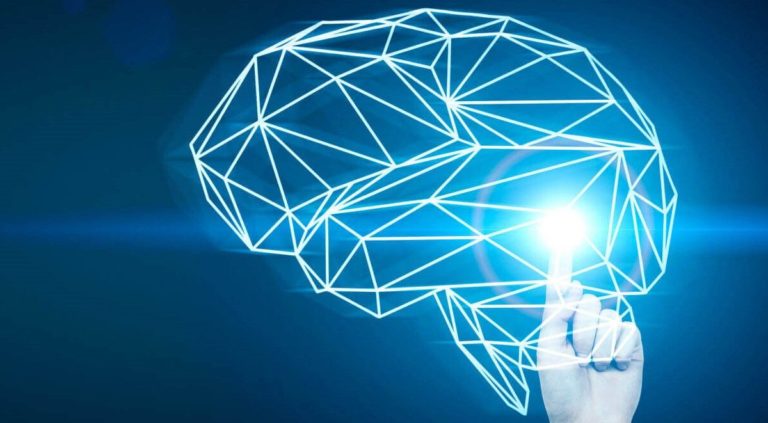
Are you too late to the party?
If you’re worried that you’ve waited too long on your machine learning investment, don’t panic. Yes, it’s true that ML adoption is in full swing. For more than a decade, companies have used the power of machine learning to improve supply chain planning efficiencies and develop optimized supply chains. Automatic model switching to improve forecast accuracy is just one of many examples of the early use of ML to continually tune the digital supply chain and optimally leverage physical supply chain network performance.
According to research published by Supply Chain Insights, “CEOs expect supply chain leaders to prepare for digital business and want to know how they intend to develop capabilities and use advanced technologies like artificial intelligence to create a flexible, agile and responsive digital supply chain.”
In short, you aren’t early, but it’s never too late. The ML market has grown rapidly, but there’s more growth and more innovation ahead. You should be part of it. Start with doing some homework and learn what’s worked for other businesses like yours. This step will help you develop an ROI model to sell the initiative internally.
#1. Understand where machine learning can have the most impact
To estimate the return on investment from leveraging machine learning, you’ll need to explore several areas of opportunity:
- Forecasting: Forecast accuracy is a top challenge for many companies and a quick-win application of ML could be the automated adoption of “best-fit” algorithms across your portfolio.
- Supply Chain Optimization: Another high-value opportunity of ML is continually analyzing the state of your digital supply chain and automatically tuning planning parameters to meet customer requirements while maximizing company objectives.
- Multi-Echelon Inventory Optimization (MEIO): Using the latest demand and supply information, machine learning can enable continuous improvement in your company’s ability to meet a desired customer service level with the lowest inventory investment.
- Automation: Automate manual data efforts and processes, leaving more time for analysts to work on value-adding activities. Improving supply chain team efficiency is key in today’s tight labor market.
- Risk mitigation: Detect problems earlier and gain a sustainable competitive advantage by proactively addressing potential disruptions.
- New product/service development: Leverage new data sources to analyze phrases and market sentiment to develop more successful new products and services that provide stronger sales and higher profits.
- Stay competitive: Your competition is likely investing in machine learning.
#2. Build the team; build experience
The right culture, organizational structure and skill sets are essential to realizing the benefits of a machine learning investment. Look for:
- Executive support – this plays an important role in building both the organizational power and vision for ML capabilities. Engage executives with business benefits, not the merits of “yet another IT project”.
- Machine learning subject matter experts with a diverse set of skills including communication and influence to build support for ML across an organization.
- Supply chain and business analysts who understand business needs, can assess the impact of changes, can determine the appropriate response and can communicate recommendations clearly.
- Data scientists who can gather, analyze and interpret complex data used in business decision-making.
- Artificial intelligence specialists to work on systems that gather information and formulate recommendations that automatically act.
#3. Choose the right digital supply chain platform
Does your supply chain platform support your plans to harness machine learning? Here are a few capabilities that your supply chain planning platform needs:
- Process automation to free up resources to focus on strategic activities
- Advanced visualization to support all your analysis requirements
- Support for all levels of analytic maturity from descriptive through to cognitive
- In-memory processing for robust analysis and fast response times
- Cloud-based for fast deployment, security, reliability and scalability
- Configurable user interface for cross-functional analysis requirements
- Data warehousing for consistent, harmonized and managed data across your entire supply chain.
Now, stop waiting and get started. Do a little research, build your ROI case and your team, implement the right platform and course correct as needed. A year from now, you should look back on 2020 as the year you made great progress implementing machine learning to boost performance across your supply chain.
Further reading:
The Top Five Benefits of Using Machine Learning for Demand Forecasting