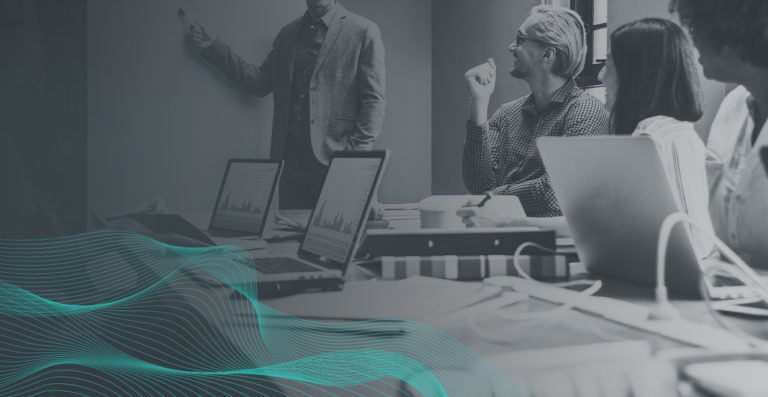
A frequent conversation on forecasting best practices among demand planners is the growing concern over the use of “one number.” From sourcing and production to logistics to marketing, most companies have long relied on a single foundational key performance indicator (KPI) to dictate how they fulfill their customer needs. But is using one-number forecasts too suspect given today’s growing availability, visibility, and use of big data, advanced analytics, and intelligent algorithms?
When it comes to getting ahead of changes in customer behavior, economic conditions, and market dynamics, there is never just one number that gives every business function the same point of view. The intent of this traditional approach may be uniformity of thought and collaborative action. But it fails to incorporate the natural distinctions across a spectrum of scenarios that warrant different decisions at different times, across different functions, across different product families and across different distribution channels to drive profitable growth strategies and margins.
The Complexity and Limitation of That One Number
A single number can mean different things to different functions within the organization. The finance team, for example, may have a revenue outlook that indicates increasing profitability, while a sales team watching its pipeline activity build up could perceive the same figure as either limiting or promising.
In essence, using one number to measure future demand loses the value of dimensionality in the data and the business process, arbitrarily forcing an organization to reach a consensus that ignores other realities. This one shortcoming is perhaps one of the most obvious for this forecasting metric.
By discounting the impact of uncertainty, businesses base their decisions on incomplete and imperfectly reliable data. The evolving best practice is to educate and empower the organization to reconcile KPIs with the realization that one-number forecasts will never be enough information to make consequential decisions.
Pragmatism Outperforms Fuzziness
Consumer-oriented businesses are characterized by various demand patterns influenced by economics, promotions, market segment, and the convenience of e-commerce and direct-to-consumer channels.
When developing a forecast for consumer industries, the underlying logic (math) needs to be multivariate and adjust over time in an autonomous manner.
Take, for example, food and beverage brands known for introducing, proliferating, and rationalizing SKU varietals. There’s a short window of opportunity between the accurate forecasting and actual consumption of a unique SKU. However, while racing to get as close as possible to the consumer, these companies still need a firm handle at a product-family level to plan their assets and network 12 to 36 months ahead and beyond.
For commodities, prescriptive capabilities – such as sensing applications, hybrid algorithms, and cause-and-effect analytics – are critical to respond to demand strategically and improve the outcome of promotions. At Logility, we have seen customers use this tactic to reduce missed sales by 50% while decreasing safety stock and overall inventory holdings in their warehouses.
In comparison, enterprises with mature products purchased weekly or monthly could benefit from statistical forecasts that are auto-tuned to benchmark operational performance. This enhancement increases visibility into trends and consumer behavior to the point where demand variances become more insightful and predictions are more accurate and trusted.
In either case, using one number is no more a complete predictor of demand. The consequences of inaccurate demand forecasting are just too risky to make decisions based on any degree of fuzziness.
The Connection Between Demand Variance and Real-Time Dynamics
Every product category is impacted by one or more causal variables. As those factors strengthen and weaken over time, depending on the forecasting cycle and the forecasting horizon, businesses can identify trends that can help improve demand forecasting.
Across the consumer products industry, different variables impact certain segments in various ways. In the Food and Beverage (F&B) industry, food purchasing is often affected by seasonal buying habits, price and promotion sensitivities, and distribution performance in ensuring a quality product reaches supermarket shelves. In the Retail, Footwear and Apparel (RFA) sector, regional preferences, economic growth and contraction, social fashion sentiment, competitors’ marketing spend, and new product introductions could influence demand for specialty fabrics used to produce high-performance and weather-resistant sportswear.
Nevertheless, avoiding this one-number forecasts fallacy requires companies to leverage KPIs that better capture their specific risks and opportunities while understanding sensitivities within the business that can change those metrics. The key is knowing how to improve business results with systematic advancement in predictive demand management.
The lesson learned about one-number forecasts is clear: the broad spectrum of demand conditions requires a unique approach to demand management. This goal can be achieved by focusing on multivariate KPIs, leveraging a range of enterprise-wide data, and collaborating on how to best leverage the data to improve decision-making. Meanwhile, businesses should also automate data processes, improve the monitoring and reporting of business results, and establish a connection between predictive accuracy and bottom-line outcomes.
While the means to achieve an accurate demand plan varies, this outside-in approach is pragmatic enough to empower organizations with more reliable processes and trusted decision-making to move the business forward – together.
Strategic Thinking Goes Beyond a Figure
If there was ever a time to rethink how businesses forecast demand and respond based on that insight, it would be now. Current economic circumstances and respondent consumer behavior have increased uncertainty and have challenged the status quo, increasing the risk of lower revenue and lost market share. And frankly, one number could never capture it all – especially as quickly as things change nowadays.
Fortunately, data availability and analytics capabilities can help create forecasting models that business functions need to make decisions with greater confidence, while considering every dimension that impacts demand. And when businesses invest in these tools, they are well-positioned to climb the leaderboard, possibly surpassing comparable competitors along the way.
Learn more about improving the quality and precision of your forecasts here.