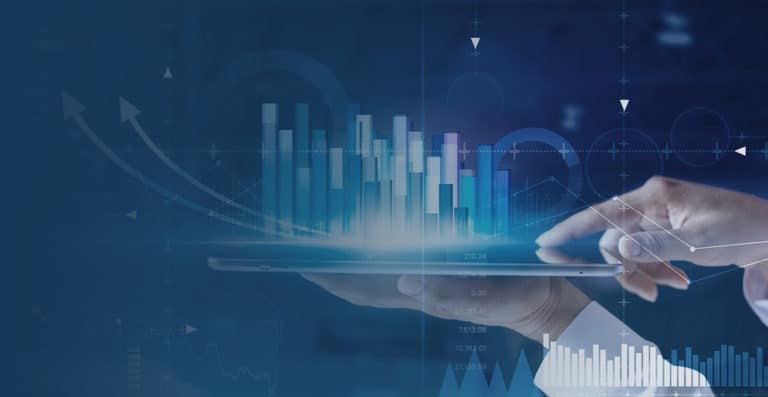
Now and then we hear from planners who claim immunity to the consistent clamor from today’s always-on global markets. A typical rebuttal might be something like, “Causal forecasting solutions sound great, but it’s not applicable to us. My company’s planning function isn’t disrupted by irrelevant inputs because we base replenishment on shipments, some seasonal adjustments and a dash of gut feel, nothing more.”
This approach is not sustainable. The race is on to deploy technologies such as machine learning and AI that are needed to convert massive, complex and disparate sets of downstream market data into insights that can improve execution across the enterprise. You can be proactive and use a causal forecasting solution to leverage data you already own, model additional data sources that could help explain demand variability… or do nothing.
What Exactly Is Causal Forecasting?
First, what it’s not is a replacement for demand forecasting. In fact, it enhances basic demand forecasting solutions by leveraging machine learning and advanced analytics to provide more insight to improve forecast quality and demand response. Causal forecasting resides between mid- and long-range planning (typically the realm of time-series planning methods) and extremely short-term demand sensing technology (“What should I ship today?”).
Logility’s causal forecasting solution is an enterprise application for merging all relevant demand signals into a single source of truth and performing predictive and visual analytics that improve forecast quality and allow a business to take impactful action ahead of the demand curve. As such, causal forecasting is much more than basing inventory positions and replenishment schedules on shipment data. Rather, it is about leaning into complexities and understanding cause and effect, not playing it safe at the expense of margin.
Users of causal forecasting solutions quickly learn that it’s about more than forecasting trends and seasonality; it’s about identifying and measuring market signals, then using those signals to shape future demand. Imagine understanding market dynamics well enough to move beyond marginally effective reactions to actually influencing outcomes!
The Benefits Offered by Causal Forecasting
In short, forecast reliability and consensus planning will improve as a result of clearly understanding how market forces correlate with changes in true demand. Causal forecasting solutions provide visibility and collaboration tools to create direct line-of-sight to real demand from customers as it happens, using this as vital intelligence to be fed back up through the supply chain.
Let’s apply it to a real scenario. You discover that during a given period your company’s sales into the retail channel are significantly higher than sales reported using POS data. Ultimately, the problem is a combination of an overly optimistic forecast and the wrong product mix. Inventory increased as overall customer service levels fell, and margins are set to erode as product is put on clearance.
To avoid this happening, a deeper understanding of causal factors is needed. For example, a planner using Logility’s causal forecasting solution to explore variability and causal relationships among stores, products, territories, inventory, raw material price fluctuations, pricing, promotions, competitive activity and consumer sentiment would discover that several brand extension launches were hindered by competitive activity, negative reviews on social media, raw material shortages and shipping delays. The planner could then immediately model adjustments and commit an improved forecast back to the master demand planning system.
Some Things to Bear in Mind
You may have determined already that causal forecasting works best in certain environments and does present some challenges. Industries such as Fast-Moving Consumer Goods (FMCG), Retail, Pharmaceuticals, High Tech and Chemical align well because they tend to share the same characteristics: inventory-driven, consumer-centric, price sensitive, with a reliance on global supply chains, and highly influenced by external factors like commodity prices. And they often share similar business and operational goals: cycle-time reduction, improved fill rates, improved customer service, better cash flow, elimination of over/under stocks, and waste reduction.
As for challenges, before you can have consistent access you need a data storage management strategy to ensure all relevant data can be gathered, housed, structured and blended appropriately. From there, causal forecasting solutions allow users to explore multiple best-fit causal models and discover patterns of “explained variance” in the data. Powerful visualization tools allow users to understand the impact of what-if simulations. The final step is integration back to your master demand planning systems.
In summary, causal forecasting helps you aggregate the data you have with the data you need to improve the accuracy of your forecasts.
Learn how causal forecasting helps predict eCommerce demand and can improve near-term forecast accuracy – Insights-Driven eCommerce Forecasting